Briefing Paper #404
Abstract
Estimates made by the Environmental Protection Agency of the likely employment effects of a proposed rule (the Clean Power Plan or CPP) mandating reductions in greenhouse gas emissions from existing power plants are likely incomplete. These estimates undercount both positive and negative influences on employment. This paper provides a comprehensive overview of the channels through which the mandated emission reductions may lead to employment changes, both positive and negative. It finds that the CPP is likely to lead to a net increase in of roughly 360,000 jobs in 2020, but that the net job creation falls relatively rapidly thereafter, with net employment gains of roughly 15,000 jobs in 2030. This paper also provides comparisons of the composition of employment in job-gaining versus job-losing industries. While workers in job-losing industries are less likely to have four-year college degrees, jobs in these industries are far less likely to be low-wage than in the overall economy, or in job-gaining industries. Workers in job-losing industries are also substantially more likely to be represented by a union. The characteristics of employment in job-losing industries, as well as the likely geographic concentration of gross job losses in poorer states, is likely to lead to transition challenges for workers and communities in responding to the CPP. This suggests the potential for a key role for federal assistance and complementary policies to aid these groups.
Introduction and summary of findings
In June 2014, the Environmental Protection Agency (EPA) issued a proposed regulation that instructs states to develop plans to reduce greenhouse gas emissions from existing fossil fuel–fired electric generating units (EGUs). The Clean Power Plan (or CPP) calls for the emission limits to be met by 2020.
This rule is the most substantial U.S. regulatory undertaking aimed at mitigating global climate change. In 2007, the Supreme Court ruled that greenhouse gas emissions are covered by the 1970 Clean Air Act’s definition of an air pollutant, and that the EPA must determine whether these emissions cause or contribute to air pollution that may be reasonably anticipated to endanger public health or welfare. Legislative efforts to mitigate greenhouse gas emissions passed in the House of Representatives in 2009, but failed to gain a vote in the Senate, despite widespread recognition that they likely had majority support. Passage of such legislation to mitigate greenhouse gas emissions would almost certainly have kept the proposed EPA rule from moving forward.
Although the economic, health, and environmental effects of the proposed rule are significant, this paper will focus on just one narrower effect: potential impacts on employment. Despite the fact that jobs and employment growth are among the smaller outcomes of the proposed rule, they tend to garner outsized attention in debate over the rule—as does the jobs impact of most environmental legislation. In the Regulatory Impact Analysis (RIA) that accompanied the release of the proposed rule, the EPA provided preliminary estimates of some of the rule’s direct impacts on U.S. employment. This paper aims to build and improve upon the EPA estimates and provide a comprehensive accounting of how the proposed rule is likely to affect U.S. employment. The key findings of this paper are:
- The proposed rule will generate both gross job gains and gross job losses, with the sum of these being a net job gain in each of the three years surveyed. The EPA estimates that in 2020 investments in energy efficiency and construction of new generating capacity (both natural gas and renewables) will boost direct employment by roughly 120,000 jobs while reduction in coal-fired electricity generation will lead to the direct displacement of roughly 24,000 jobs. These direct job gains and displacements will hence lead to a direct net job gain of roughly 96,000.
- Each job directly created or displaced will create substantial ripple effects in other sectors. This paper aims to assess the “employment multiplier” associated with each of these first-round job impacts estimated by the EPA, accounting for the effect of these direct job gains and losses on supplier jobs, induced (re-spending or “Keynesian” effect) jobs, and public-sector jobs. Jobs in losing industries tend to have slightly higher employment multipliers than jobs in gaining industries, but when these effects are factored in, the net number of jobs supported in 2020 is roughly 360,000.
- In the longer term (through 2030), in part because employment multipliers are slightly higher in losing industries, the CPP’s indirect effects actually reduce the net number of jobs supported below the EPA estimates of net direct job creation, yielding roughly 24,342 net jobs supported by that year.
- Another channel through which the CPP could affect employment that is missing from EPA estimates concerns the effect of electricity price increases. The CPP is estimated to raise electricity prices by 5 percent in 2020, and by smaller amounts in 2025 and 2030. In the longer term (2025 and 2030), there is little evidence that these price increases will measurably impact employment. In the near term (2020), if consumers and businesses fail to anticipate or properly plan for these price increases, employment could fall by between 25,000 and 150,000 jobs.
- The labor force characteristics of jobs displaced and jobs supported following the CPP are quite different. Jobs displaced are more likely to be unionized and skew even more toward male workers than jobs supported. Jobs displaced are also less likely to be low-wage and more likely to be high-wage than jobs supported, even though jobs displaced are more likely to be filled by workers without a four-year college degree.
- Gross job losses are likely to be geographically concentrated, raising the challenge of ensuring a fair transition for workers in sectors likely to contract due to the CPP.
The first section of the paper describes the possible theoretical channels through which the CPP may affect employment. Subsequent sections provide an empirical assessment of each channel, followed by a sum of the effects to provide an overall estimate of the employment changes likely to be spurred by the CPP. This estimate includes gross job gains, gross job losses, and net changes (the sum of gross positive and negative changes). Finally, the paper examines job quality differences between gross job gains and gross job losses.
Channels through which the CPP may affect employment
The CPP mandates emission reductions on a state-by-state basis. By setting an overall state target, however, it leaves states many margins of adjustment along which to realize these emission reductions. For example, states could mandate that a share of overall electricity generation come from non-emitting sources. Or they could provide incentives for businesses, utilities, and households to make investments in energy efficiency. There is even the possibility of states joining together to form a regional cap and trade system that only allows utilities to emit greenhouse gases after purchasing a marketable permit to do so. Given this flexibility in how states respond to the CPP, there is great uncertainty in the precise economic outcomes that will be driven by the rule’s implementation. For the purposes of this paper, I follow the economic modeling undertaken by the EPA in its RIA and translate its economic projections (including preliminary employment projections) into comprehensive measures of employment changes.
Economic margins of adjustment
The EPA’s RIA identifies a number of margins of economic adjustment as likely to be most important to states to meet the emissions reduction guidelines. In the near term, electricity production from coal-fired electrical generating utilities will fall, and output by natural gas-fired power plants will increase. Construction of new electricity generation capacity from renewables (mostly wind and solar) will be front-loaded during the first 10 years of the rule, accelerating additions of renewable generating capacity. Solar and wind power will then replace some of the declines in coal-fired generation, particularly in the medium term (more than five years out). Energy efficiency investments will also be accelerated by the rule. These efficiency investments in homes, businesses, and industry will allow electrical generation to fall significantly relative to the non-CPP baseline by 2030. Examples of such energy efficiency investments include the purchase of more efficient home appliances and upgrading of insulation in residential homes; the optimization of heating, ventilation, air-conditioning systems, and electrical lighting in commercial buildings; and process optimization through modern instrumentation and control systems in the industrial sector.
Further, the sum of these effects is expected to raise electricity prices, particularly in the near term. The efficiency investments will, however, sufficiently dampen the demand for electricity quantities to lower overall household electricity spending by the end of the period described in the EPA RIA.
Employment margins of adjustment
Employment changes will follow directly from these economic margins of adjustment. A number of channels will lead to employment reductions. For example, retirement of coal-fired electrical generating capacity will lead to losses in operations and maintenance employment at existing coal-fired power plants. These effects show up in both short- and longer-run horizons examined in the RIA. The switch from coal-fired generation will lead to a reduction in demand for coal, and subsequent significant declines in both the short and long term for coal mining jobs. Increases in energy prices will spur employment responses, including demand-side reductions in spending, as households facing higher electricity bills (at least in the short run) curtail spending on non-energy goods. There will also be supply-side reductions as the (slight) decline in real wages spurred by rising energy prices affects labor supply decisions. Finally, there may be responses related to international competitiveness, as higher domestic energy prices affect the cost of industrial production in the United States.
Conversely, a number of changes spurred by the CPP will lead to employment gains (or at least to no losses) in both the near and longer term. For example, investments in energy efficiency will lead to employment increases in all time horizons. Short-term investments in heat rate improvement of existing fossil-fuel power plants will spur employment in the near term without reducing employment in the longer term.
Some of these margins of economic adjustment to the CPP have different employment impacts depending on the time horizon. For example, construction of new natural gas generation capacity boosts employment in the short run, but reduces employment in the longer run, as jobs associated with planned EGU expansions from natural gas are pulled forward in time by the rule. In the near term, this implies increases in construction jobs for building this new capacity, but some of this short-term employment boost comes at the expense of construction in the medium and longer term. Similarly, construction of new renewable generation capacity creates employment growth in the short term, but reductions in the medium and longer term as these jobs are pulled forward relative to the non-CPP baseline. Finally, operations and maintenance jobs at natural gas power plants will rise slightly in 2020 and 2025 and fall slightly in 2030.
Finally, each of these channels will in turn spur indirect effects. The indirect effects tracked in this paper will include: supplier jobs, induced (Keynesian) re-spending jobs, and public-sector jobs supported through tax revenue. The sections that follow will provide an empirical estimate of the effects (including indirect effects) of each of these channels.
Direct employment effects: Translating changes by economic activity into industry changes
This section will first report the estimates on direct employment effects contained in the EPA’s RIA, and will then assign these employment effects specific industry codes that can be used as inputs into employment requirement matrices in order to undertake the analysis of indirect effects included in later sections of the report.
The RIA essentially provides four different estimates (or scenarios) for each of these flows in every year. The RIA estimates effects stemming from a “state-only” or single state approach and a “regional” approach to meeting emissions targets. The CPP provides the option for states to collectively meet combined (regional) emissions targets. This may alter the margins of adjustment for meeting emissions guidelines as compared with a single-state approach. The RIA also provides two different options for the level and pace of emissions reductions that states must meet. One of these options is recommended by the EPA; the second is offered (and public comment is invited). In what follows, I average the outcomes estimated in the RIA in the four different scenarios (two emissions guidelines that can be met by either single-state or regional action). Because the differences in outcomes stemming from the four different scenarios are quite small, this averaging approach does not compromise the overall findings.
The main driver of these direct effects on employment is simply the change in electricity generation: both overall and by type (summarized in Table 1). Throughout this paper, economic impacts (whether on electrical generation, prices, or job flows) of the CPP will be expressed relative to a baseline estimated by the EPA regarding the likely path of these variables if the CPP is not implemented. Relative to this non-CPP baseline projection for future electricity generation, the CPP leads to an 18.6 percent decline in coal-fired electricity generation by 2020, and a 26.1 percent decline by 2030. Renewables, conversely, rise by 6.4 percent by 2020 relative to the non-CPP baseline. By 2030, however, renewable generation is just 1.7 percent above the projected baseline. Natural gas generation rises by 14.6 percent relative to the non-CPP baseline by 2020, but by 2030 actually falls 5.7 percent. Besides the decline in coal-fired generation, the most striking finding in Table 1 is the decline in total generation, which is essentially a reflection of energy efficiency investments. Relative to the baseline, total generation falls 2.8 percent by 2020 and 11.3 percent by 2030.
Electricity generation by source, baseline and under the Clean Power Plan, in 2020, 2025, and 2030
Totals (megawatts) | Shares | ||||||
---|---|---|---|---|---|---|---|
Baseline | Post-CPP | Change | % Change | Baseline | Post-CPP | Ppt change | |
2020 | |||||||
Coal | 1665 | 1355 | 310 | -18.6% | 39.5% | 33.0% | -6.4 |
Natural gas | 1159 | 1328 | -169 | 14.6% | 27.5% | 32.4% | 4.9 |
Nuclear | 817 | 817.25 | -0.25 | 0.0% | 19.4% | 19.9% | 0.6 |
Hydro | 280 | 281.5 | -1.5 | 0.5% | 6.6% | 6.9% | 0.2 |
Non-hydro renewables | 299 | 318.25 | -19.25 | 6.4% | 7.1% | 7.8% | 0.7 |
Total | 4220 | 4100 | 120 | -2.8% | 100.0% | 100.0% | 0.0 |
2025 | |||||||
Coal | 1702 | 1315.25 | 386.75 | -22.7% | 38.7% | 32.1% | -6.6 |
Natural gas | 1263 | 1340 | -77 | 6.1% | 28.7% | 32.7% | 4.0 |
Nuclear | 817 | 817.25 | -0.25 | 0.0% | 18.6% | 19.9% | 1.4 |
Hydro | 280 | 281.75 | -1.75 | 0.6% | 6.4% | 6.9% | 0.5 |
Non-hydro renewables | 335 | 344 | -9 | 2.7% | 7.6% | 8.4% | 0.8 |
Total | 4397 | 4098.25 | 298.75 | -6.8% | 100.0% | 100.0% | 0.0 |
2030 | |||||||
Coal | 1668 | 1232.5 | 435.5 | -26.1% | 36.7% | 30.5% | -6.1 |
Natural gas | 1455 | 1372 | 83 | -5.7% | 32.0% | 34.0% | 2.0 |
Nuclear | 797 | 796.5 | 0.5 | -0.1% | 17.5% | 19.7% | 2.2 |
Hydro | 280 | 280.5 | -0.5 | 0.2% | 6.2% | 6.9% | 0.8 |
Non-hydro renewables | 350 | 356 | -6 | 1.7% | 7.7% | 8.8% | 1.1 |
Total | 4550 | 4037.5 | 512.5 | -11.3% | 100.0% | 100.0% | 0.0 |
Note: The sum of totals and shares columns may vary slightly from change columns due to rounding.
Source: EPA Regulatory Impact Analysis of Clean Power Plan (2014). The table's estimates average two emissions targets and options to meet them with state or regional plans.
The projected change in total electrical generation leads to corresponding changes in employment flows that are directly estimated by the RIA. The directly estimated employment changes by category are summarized in an appendix in Table A1. Before presenting these findings on the direct employment flows, however, it is important to be specific about how these are expressed.
Again, each employment impact is relative to what would occur in the EPA’s non-CPP baseline. Relative to this baseline, the EPA estimates a change in coal extraction in 2020, 2025, and 2030. In 2020, coal extraction employment is down 12,600 jobs relative to the non-CPP baseline. This means that employment in coal mining is lower by 12,600 jobs than would otherwise be expected in that year because of the CPP. In 2025, coal extraction employment is down 15,300 jobs relative to the baseline. This does not mean that coal-mining employment is lower by 15,300 jobs in 2025 than it was in 2020, but that the estimate is relative to that in a non-CPP world. Further, one cannot add the 2020 and 2025 estimates together and say that coal-mining employment is reduced by 27,900 in 2025 due to the CPP. One can infer that the effect of the CPP on coal-mining employment between 2020 and 2025 is a reduction of 2,700 (the difference between 15,300 and 12,600). Qualitatively, this means that the bulk of the effect of the CPP on coal-mining extraction occurs before 2020, and that the rule’s drag on coal extraction employment thereafter is less intense (although it does still grow).
Identifying the specific industries affected by the EPA employment estimates
Indirect employment effects associated with the direct employment consequences identified in the RIA lean heavily on the use of input-output (or employment requirements) matrices to identify supplier jobs associated with direct employment changes. This approach necessitates categorizing the direct employment losses identified in the RIA according to the 195 industrial sectors covered by the employment requirements matrices (ERMs) that are available from the Bureau of Labor Statistics (BLS). The EPA analysis of employment changes by economic activity detailed in Table A1 can be translated into employment changes occurring in the industrial sectors in the ERM. The exact mapping of economic activity identified by the EPA employment estimates to an industrial classification is provided in Appendix C. The outcome of this mapping is summarized in Table 2, which presents employment changes by gaining and losing industries separately for 2020, 2025, and 2030, as well as the net industry employment effects. I discuss the BLS ERM in greater detail in the next section.
Direct employment changes under the Clean Power Plan by industry in 2020, 2025, and 2030
Gains | Losses | Net | |||||||||
---|---|---|---|---|---|---|---|---|---|---|---|
2020 | 2025 | 2030 | 2020 | 2025 | 2030 | 2020 | 2025 | 2030 | |||
Oil and gas extraction | 5,050 | 2,700 | 0 | 0 | 0 | 2,000 | 5,050 | 2,700 | -2,000 | ||
Coal mining | 0 | 0 | 0 | 12,600 | 15,300 | 17,300 | -12,600 | -15,300 | -17,300 | ||
Electric power generation, transmission and distribution | 0 | 0 | 0 | 11,663 | 20,425 | 24,300 | -11,663 | -20,425 | -24,300 | ||
Construction | 16,160 | 3,203 | 1,313 | 0 | 0 | 0 | 16,160 | 3,203 | 1,313 | ||
Plastics product manufacturing | 953 | 0 | 0 | 0 | 345 | 129 | 953 | -345 | -129 | ||
Machine shops: hardware | 1,389 | 0 | 0 | 0 | 503 | 188 | 1,389 | -503 | -188 | ||
Fabricated metal | 2,104 | 0 | 0 | 0 | 4,633 | 5,977 | 2,104 | -4,633 | -5,977 | ||
HVAC equipment manufacturing | 20,573 | 17,269 | 17,440 | 0 | 0 | 0 | 20,573 | 17,269 | 17,440 | ||
Engine, turbine and power transmission equipment manufacturing | 12,970 | 0 | 0 | 0 | 5,107 | 8,048 | 12,970 | -5,107 | -8,048 | ||
Machinery manufacturing | 2,937 | 0 | 0 | 0 | 1,064 | 398 | 2,937 | -1,064 | -398 | ||
Communications equipment | 551 | 763 | 771 | 0 | 0 | 0 | 551 | 763 | 771 | ||
Electric lighting manufacturing | 30,388 | 42,114 | 42,530 | 0 | 0 | 0 | 30,388 | 42,114 | 42,530 | ||
Household appliance manufacturing | 2,624 | 3,637 | 3,673 | 0 | 0 | 0 | 2,624 | 3,637 | 3,673 | ||
Electrical equipment manufacturing | 4,164 | 3,342 | 3,695 | 0 | 0 | 0 | 4,164 | 3,342 | 3,695 | ||
Other electrical equipment and component manufacturing | 1,627 | 0 | 0 | 0 | 589 | 220 | 1,627 | -589 | -220 | ||
Design services | 1,152 | 0 | 0 | 0 | 4,288 | 5,848 | 1,152 | -4,288 | -5,848 | ||
Management, scientific and technical consulting services | 8,113 | 0 | 0 | 0 | 0 | 0 | 8,113 | 0 | 0 | ||
Scientific research and development services | 1,945 | 0 | 0 | 0 | 704 | 263 | 1,945 | -704 | -263 | ||
Services to buildings and dwellings | 7,238 | 10,031 | 10,130 | 0 | 0 | 0 | 7,238 | 10,031 | 10,130 | ||
Total | 119,938 | 83,059 | 79,552 | 24,263 | 52,959 | 64,672 | 95,675 | 30,100 | 14,880 |
Note: The table categorizes the job losses into the industrial sectors covered by the Bureau of Labor Statistics' Employment Requirements Matrices (ERMs).
Source: EPA Regulatory Impact Analysis of Clean Power Plan (2014) and author's analysis described in the text
Indirect employment effects
By taking the EPA’s estimates of first-round employment changes spurred by the CPP and calculating their indirect job impacts, this paper adds to the CPP’s impact assessment. In particular, because jobs in different industries can have very different levels of indirect jobs associated with them, the EPA estimates of net job creation and displacement could be different or even change in sign from positive to negative when these indirect effects are taken into account. In this section, I estimate three separate categories of indirect job impacts that are spurred by the first-round employment changes documented in the RIA: supplier jobs, induced (or re-spending) jobs, and public-sector jobs. I label the total of these influences as the “employment multiplier.”1
Supplier jobs, materials
Supplier jobs are generally the most intuitive category of indirect employment changes. Put simply, when jobs are lost in one industry sector, the sectors that provide inputs and materials also suffer losses. Take a concrete example: When coal-mining activity shrinks, it leads to a reduction in demand for industries that provide inputs to coal mining, such as those that provide safety equipment, industrial equipment, and/or transportation equipment.
Supplier job estimates can be calculated directly from the BLS ERM. The ERM shows how many jobs are supported by $1 million in final demand in a given sector, jobs both in the sector directly satisfying the final demand as well as ones supplying inputs. For example, each $1 million in final demand for construction services supports jobs in the construction sector, but also supports jobs in concrete production, bulldozer manufacturing, and accounting services. The ERM tracks how many jobs in these supplier industries are supported by each $1 million in construction services purchased.
Because the ERM is set up in terms of dollar flows rather than job flows, translating the direct employment impacts identified by the RIA into supplier jobs requires a small manipulation. Specifically, I take the ratio of jobs supported by a given amount of spending in a sector that are supplier jobs to direct jobs, and then multiply this ratio by the number of direct jobs identified in the RIA. The estimate for supplier jobs supported by each 100 direct jobs in a given sector is calculated using the following equation:
Supplier jobs, capital services
One weakness of the BLS ERM is that it does not account for the depreciation of capital goods (plant, equipment, and structures) that is caused by production. For very capital-intensive industries—and utilities and extraction are both notably capital-intensive—this could have nontrivial impacts on jobs supported.
To correct this, I estimate the number of jobs associated with producing the capital goods that would be needed to replace the amount of depreciation associated with 100 direct jobs in an industry. First, I estimate the value of capital services used in each industry’s production. To do this, I use data from the BLS data series on multi-factor productivity (MFP), which provides data on the capital share of output (that is, the share of income generated by each industry that goes to pay owners of capital goods rather than workers). Combining industry output with the capital share of output provides an estimate of the amount of new capital goods that must be produced each period to replace this capital service flow. Essentially, capital-intensive industries will have to spend more money to replace capital services that are used up during production. Because I begin with a given number (100) of jobs (rather than output) in each industry, calculating industry output again requires a small manipulation of the data. The first expression, in parentheses, shows how output (measured in dollars) per each 100 workers in a given industry can be calculated. This output measure is then multiplied by the capital share to give the expression for depreciation (or capital service inputs) associated with each industry.
This measure of depreciation is then used to estimate industry capital demand. Based on ratios that approximately reflect the economy-wide division of aggregate capital investment to structures versus equipment, I assume that 40 percent of this total spending flows into construction to replace new structures and that 60 percent flows into equipment manufacturing to replace machinery. From here, the formula for supplier jobs to replace the depreciation involved with every 100 direct jobs in a given industry is:
Induced (or re-spending) jobs
Another category of indirect jobs concerns those that are supported by the demand that relies on the wage and salary income of direct jobs. For example, each 100 jobs in construction also support jobs in restaurants and diners where construction workers eat, grocery stores where they shop for food, and doctors’ offices where they pay for medical services.
The scale of induced jobs supported by each 100 direct jobs depends on the overall “re-spending multiplier.” Bivens (2003) reviewed evidence on this multiplier and took 0.5 as a conservative estimate of this effect. Induced jobs also depend on the relative wages of both direct and supplier industries. As an example, if automobile assembly jobs have wages that are 50 percent higher than the economy-wide average wage, this would lead to spending induced by each 100 jobs in that sector that is 50 percent higher than the economy-wide average, making the induced spending multiplier this much higher. Further, if the supplier jobs supported by automobile assembly (steel, iron, glass, etc.) pay higher-than-average wages, then this will also increase the induced spending multiplier for the automobile assembly sector.
In this paper, I index hourly wages by industry to establish an economy-wide average of one. From here, one can express the induced jobs supported by each 100 direct jobs in an industry as simply 100 times the index of average hourly wages in the industry times 0.5 (our re-spending multiplier). For supplier jobs, I multiply the (195 industries) vector of supplier jobs associated with a given 100 jobs in the direct industry by each industry’s average hourly wage index, multiply by 0.5 (the re-spending multiplier) and then sum to estimate the induced spending from supplier jobs associated with direct employment in a given sector.
Public-sector jobs
Finally, we can estimate the number of public-sector jobs (federal, state, and local) associated with each 100 direct jobs in an industry. This measure differs across industries based on the relative wage of the industry. To generate the inputs for this calculation, I multiply each industry’s hourly wage by 2,000 to express it as a full-time, full-year salary. For federal taxes, I multiply this figure by 0.2, and for state and local taxes, by 0.1. This provides a rough measure of the tax revenue supported by each job in an industry.
I then use U.S. Census Bureau data to obtain estimates of overall tax revenue and employment in federal, state, and local governments. Dividing total tax revenue by employment, I get a measure of how much tax revenue is required to support a public-sector employee in federal versus state and local government employment. I then divide the tax revenue generated by each 100 jobs in a given industry by this per employee wage bill to get a measure of public-sector employment generated.
Summing up the indirect effects of changing industry employment
Table 3 provides a summary of the indirect effects (or employment multipliers) for each of the direct industry job flows estimated by the EPA. The largest multipliers, by a considerable margin, are in the oil and gas mining sector and the utilities (electric power) sector. Large multipliers also are found in most of the manufacturing industries that receive considerable direct job flows. The overarching effect of the job multipliers is to increase the total net employment impact spurred by the direct spending flows that are likely to occur due to the CPP in the near term. In 2020, approximately 95,000 more direct jobs are generated directly through energy efficiency investments, heat rate investments, and construction of new capacity than are displaced directly from coal plants retiring early and mining jobs being lost. Further, more than 264,000 additional jobs are generated when indirect effects are considered. However, by 2030, the estimated job gains are smaller than the direct employment flows would indicate. This is largely due to two influences: First, direct job creation in later years is expected to ebb because renewable and natural gas generation investments triggered by the CPP largely represent an acceleration of investments that would have occurred eventually even in the absence of the CPP; and second, the employment multipliers of jobs in EGUs and coal mining are large, and these sectors are projected to shed jobs even in the medium and longer terms.
Employment multipliers by industry (indirect jobs supported by every 100 direct jobs) in 2020, 2025, and 2030
Direct jobs | Indirect effects per 100 direct jobs | Total (direct + indirect) jobs | |||||||||||
---|---|---|---|---|---|---|---|---|---|---|---|---|---|
Supplier jobs | Respending Jobs | Public jobs | Total | ||||||||||
2020 | 2025 | 2030 | Materials | Capital services | Direct | Indirect | Federal | State + local | 2020 | 2025 | 2030 | ||
Oil and gas extraction | 5,050 | 2,700 | -2,000 | 271.9 | 200.0 | 29.0 | 158.6 | 4.2 | 9.9 | 673.5 | 39,063 | 20,885 | -15,470 |
Coal mining | -12,600 | -15,300 | -17,300 | 89.7 | 61.9 | 60.7 | 48.6 | 2.4 | 5.8 | 269.0 | -46,495 | -56,458 | -63,838 |
Electric power generation, transmission, and distribution | -11,663 | -20,425 | -24,300 | 152.7 | 187.9 | 47.9 | 72.0 | 2.7 | 6.3 | 469.5 | -66,423 | -116,330 | -138,400 |
Construction | 16,160 | 3,203 | 1,313 | 40.8 | 32.7 | 72.0 | 21.5 | 2.1 | 4.9 | 173.9 | 44,265 | 8,774 | 3,598 |
Plastics product manufacturing | 953 | -345 | -129 | 99.6 | 24.6 | 55.9 | 50.7 | 2.4 | 5.6 | 238.8 | 3,227 | -1,169 | -437 |
Machine shops: hardware | 1,389 | -503 | -188 | 54.7 | 19.8 | 43.0 | 28.5 | 1.6 | 3.8 | 151.4 | 3,492 | -1,265 | -473 |
Fabricated metal | 2,104 | -4,633 | -5,977 | 101.7 | 18.8 | 47.1 | 52.4 | 2.2 | 5.2 | 227.5 | 6,891 | -15,174 | -19,575 |
HVAC equipment manufacturing | 20,573 | 17,269 | 17,440 | 144.8 | 54.8 | 41.4 | 75.0 | 2.6 | 6.1 | 324.7 | 87,371 | 73,338 | 74,063 |
Engine, turbine, and power transmission equipment manufacturing | 12,970 | -5,107 | -8,048 | 166.2 | 64.5 | 53.3 | 85.9 | 3.1 | 7.3 | 380.4 | 62,303 | -24,534 | -38,662 |
Machinery manufacturing | 2,937 | -1,064 | -398 | 148.7 | 29.7 | 63.6 | 76.0 | 3.1 | 7.3 | 328.4 | 12,582 | -4,557 | -1,704 |
Communications equipment | 551 | 763 | 771 | 137.7 | 72.1 | 46.8 | 76.0 | 2.7 | 6.5 | 341.8 | 2,433 | 3,371 | 3,405 |
Electric lighting manufacturing | 30,388 | 42,114 | 42,530 | 126.5 | 48.7 | 75.5 | 66.8 | 3.2 | 7.5 | 328.2 | 130,108 | 180,312 | 182,094 |
Household appliance manufacturing | 2,624 | 3,637 | 3,673 | 198.1 | 75.3 | 65.5 | 102.0 | 3.7 | 8.8 | 453.5 | 14,527 | 20,132 | 20,331 |
Electrical equipment manufacturing | 4,164 | 3,342 | 3,695 | 82.7 | 27.3 | 64.5 | 43.2 | 2.4 | 5.7 | 225.8 | 13,566 | 10,889 | 12,039 |
Other electrical equipment and component manufacturing | 1,627 | -589 | -220 | 108.9 | 35.3 | 59.2 | 56.8 | 2.6 | 6.1 | 268.8 | 6,001 | -2,174 | -813 |
Design services | 1,152 | -4,288 | -5,848 | 31.4 | 28.1 | 61.0 | 18.2 | 1.8 | 4.2 | 144.7 | 2,818 | -10,492 | -14,308 |
Management, scientific and technical consulting services | 8,113 | 0 | 0 | 47.5 | 32.1 | 47.7 | 30.2 | 1.7 | 4.1 | 163.3 | 21,364 | 0 | 0 |
Scientific research and development services | 1,945 | -704 | -263 | 80.7 | 48.7 | 46.6 | 48.3 | 2.1 | 5.0 | 231.4 | 6,444 | -2,334 | -873 |
Services to buildings and dwellings | 7,238 | 10,031 | 10,130 | 16.7 | 12.3 | 85.2 | 9.3 | 2.1 | 5.0 | 130.6 | 16,693 | 23,135 | 23,363 |
Total | 95,675 | 30,100 | 14,880 | 360,229 | 106,352 | 24,342 |
Note: Direct jobs by industry from Table 2 and Table A4. Indirect effects estimated using method described in text. The table categorizes the job losses into the industrial sectors covered by the Bureau of Labor Statistics' Employment Requirements Matrices (ERMs).
Source: Author’s analysis using data from the EPA RIA of the Clean Power Plan, BLS Employment Requirements Matrix (ERM), BLS Multifactor Productivity data series, and Census data on government spending and employment
Price effects on employment
There will also be job effects stemming from the rise in electricity prices projected to result from the new rule. On average across the four scenarios (“Option 1 and 2” and “State and Regional” approaches in the RIA), the electricity price increase by 2020 will be 5 percent, and will decline to 2.7 and 2.9 percent in 2025 and 2030, respectively. Economic theory is far from settled on how the rise in a single price in the economy will affect economy-wide employment. In this section, I provide some broad parameters about the possible impacts, and then offer some evidence from simple regressions to assess the impact of electricity price changes on employment.
In order to establish some parameters to check the plausibility of regression results, assume first that the entire 5 percent increase in electricity prices leads to no reduction in electricity demand from consumers. Multiplying this 5 percent by electricity’s share in the total economy (2.4 percent)2 translates to a 0.12 percent decline in economy-wide demand for goods and services besides electricity. That is, by having to pay 5 percent more for electricity and not adjusting their demand at all, American households would have 0.12 percent less to spend on non-electricity goods and services. Given that economy-wide consumption spending in 2013 was roughly $11.5 trillion, this implies an approximate $14 billion decline in purchasing power. Given that each job in the U.S. economy is associated with roughly $140,000 in gross domestic product, this approximate $14 billion decline in purchasing power in turn translates into an estimated 100,000 jobs that would be displaced by a demand reduction of this magnitude.3
But of course, this assumption of no demand response is extremely strong. Responsiveness of consumers to energy price increases (or the elasticity of demand for electricity) may be relatively low in the short run, but is expected to be greater than zero, and there is strong evidence that it rises sharply over time (see Maddala et al. 1997.)
A strong assumption in the opposite direction, that a 5 percent increase in the price of electricity would be met immediately by a 5 percent reduction in demand for electricity (implying an elasticity of demand of one), would suggest that there would be no overall demand effect stemming from reduced consumer spending; consumers would simply shift their spending away from electricity and toward other goods and services.
This thought experiment helps to establish some parameters for what a reasonable estimate of the employment response to an electricity price increase should be based simply on consumers’ responses. Given that consumer spending is two-thirds of the U.S. economy, the employment response due to changes in consumer spending is expected to be a large part of the total employment effects.4 Any estimates of job declines that are much larger than the high end of these rough benchmarks essentially need to be accompanied by a compelling theoretical reason for why so they are so large, since the high end of mechanical effects of higher electricity prices “crowding out” spending on other goods seems well-defined for price increases of 5 percent or less.
Regression analysis
In this section, I undertake two methods of regression analysis to assess the impact of higher electricity prices on overall employment. First, I use a vector autoregression of total nonfarm payroll employment on changes in electricity prices (following Killian 2008). By ordering electricity prices first, and making the assumption that employment changes in a given month do not affect electricity price changes in that same month, the results can be interpreted as the causal effect of electricity price changes on employment. Second, I assemble a panel dataset of states from 1976 to 2013 to test how changes in electricity prices correlate with employment changes. For this set of regressions, I follow Deschenes (2012).
Vector autoregression estimates
For the vector autoregression test, I use data on nonfarm payroll employment and electricity price data from the consumer price index (CPI), both from the BLS. I run a vector autoregression with electricity prices ordered first. To assess the effect of higher electricity prices on employment, I simulate the effect of an electricity price shock. Figure A shows the results of this “impulse response function,” showing how employment responds to a one standard deviation shock to electricity prices.
Response of employment to a one standard deviation change in electricity prices, results from vector autoregression
1 | 2 | 3 | |
---|---|---|---|
0 | -0.00072% | -0.01550% | 0.01410% |
1 | 0.00088% | -0.01430% | 0.01610% |
2 | -0.00490% | -0.02070% | 0.01100% |
3 | -0.01510% | -0.03130% | 0.00100% |
4 | -0.00810% | -0.02040% | 0.00430% |
5 | -0.01190% | -0.02460% | 0.00082% |
6 | -0.01000% | -0.02110% | 0.00110% |
7 | -0.00910% | -0.01920% | 0.00096% |
8 | -0.00830% | -0.01740% | 0.00074% |
9 | -0.00720% | -0.01510% | 0.00074% |
10 | -0.00630% | -0.01330% | 0.00066% |
11 | -0.00550% | -0.01160% | 0.00063% |
12 | -0.00470% | -0.01000% | 0.00061% |
13 | -0.00400% | -0.00870% | 0.00058% |
14 | -0.00350% | -0.00750% | 0.00055% |
15 | -0.00290% | -0.00640% | 0.00053% |
16 | -0.00250% | -0.00550% | 0.00050% |
17 | -0.00210% | -0.00470% | 0.00048% |
18 | -0.00180% | -0.00410% | 0.00045% |
19 | -0.00150% | -0.00350% | 0.00042% |
20 | -0.00130% | -0.00300% | 0.00039% |
21 | -0.00110% | -0.00260% | 0.00037% |
22 | -0.00094% | -0.00220% | 0.00034% |
23 | -0.00080% | -0.00190% | 0.00032% |
24 | -0.00067% | -0.00160% | 0.00029% |
Note: Impulse response function from VAR regression as described in the text. Electricity prices are ordered first, imposing the requirement that prices are not affected by employment.
Source: Monthly employment data from the BLS CES series, monthly electricity price changes from the BLS Consumer Price Index (CPI) series
The data show a clear pattern of a quick decline in employment that converges back toward zero effect. The magnitudes (.015 percent, multiplied by the 2014 work force of roughly 140 million) suggest an employment decline of nearly 20,000 (0.015 percent, as indicated on the figure) after three to five months, and then a fadeout of more than 90 percent of the effect within a year (with the remaining negative effect no longer statistically significant). A one standard deviation shock to electricity prices in this data is 4 percent, so I multiply the employment decline from the impulse response function by five fourths to estimate the employment impact of a 5 percent increase in electricity prices generated by the CPP, giving a final point estimate of 25,000 jobs displaced by higher electricity prices.
State panel regressions
The state/year panel results are summarized in Appendix Table A4. This table shows the results of a regression that uses the log of the level of state employment on the log of electricity prices across states. The employment data come from the BLS, while state-level electricity price data come from the State Energy Data Service (SEDS). This electricity price used is the average retail price for all end-users in each year between 1979 and 2012.
The preferred specification is shown in column 3 of Table A4, which controls for time and year fixed effects, state-level time trends, and the unemployment gap (which is important to include as it seems to be absorbing some employment variation not controlled for in the state, year, and time-trend dummy variables). The data cover the period from 1979 to 2012. For this specification, the coefficient of employment on energy prices is -0.017. This implies that a 10 percent change in electricity prices reduces employment by 0.17 percent, or that a 5 percent increase in electricity prices (as forecast by the CPP RIA) will reduce employment by 0.085 percent, or by just under 100,000 jobs.
As noted by Deschenes (2012), this is best interpreted as the short-run effect on employment of unanticipated increases in electricity prices. Electricity price changes that are fully expected and take some time before implementation are expected to be significantly smaller.
Greater losses result if the sample is cut off in 2008 (as is done in columns 4 to 6 of Table A4). Here, the coefficient estimates suggest job losses of more than 150,000 in the specification (column 6) that includes all other controls. It is unclear why including the latest five years of data changes the results to such a great extent, but it is regarded as more robust to proceed by including more data rather than less.
Finally, regressions that used state/industry cells as the unit of analysis are reported. The employment data let us examine 13 separate industrial sectors within states. Despite the larger sample size, the overall coefficient on state/industry employment in this larger panel never achieved statistical significance. Later sections focus just on manufacturing employment across states and do find significant and disproportionate job losses in this sector that are correlated with electricity price differences.
Combining the VAR and state-panel regression results
The two different regression techniques provide results that span most of the plausible variation identified in the introduction to this section. The results range from 20,000 to 100,000 in the preferred specifications, with 150,000 in the panel regression with the time period truncated in 2008.
Lacking any better alternative, I average the results provided by each method to establish the point estimate of the employment impact of higher energy prices. This method gives a net decrease of 75,000 jobs (the average of 25,000 and 125,000). Further, given the sharp fadeout of negative employment impacts in the vector autoregression, the interpretation of state panel regression results as measuring the responsiveness of employment to unanticipated short-run electricity price changes, and the evidence that the long-run elasticity of demand with respect to electricity prices is much larger than the short-run elasticity, I can only be confident about these negative price effects for the first year examined in the RIA–2020.
Total net employment impacts
Table 4 provides the final tally on employment impacts, showing gross gains and gross losses by each employment channel: direct effects, indirect effects, and price effects, in 2020, 2025, and 2030.
The negative price effects are not large enough to swamp the positive net effect of tallying the direct and indirect job flows. The key driver of these positive net effects is the large increase in energy efficiency investments. These investments are large in direct scale (accounting for more than half of the total direct gross gains in 2020, and accounting for essentially all of the gross gains in 2025 and 2030), and also tend to have higher than average employment multipliers. These energy efficiency investments also implicitly drive a large part of the generation response, as an overall decline in electricity use of roughly 11 percent is spurred by the rule relative to the non-CPP baseline in 2035.
In 2020, net employment changes resulting from the rule total to an employment gain of more than 285,000 jobs. This net gain drops off rapidly in 2025 and 2030 but remains positive, assuming that price effects are no longer significantly affecting employment in 2025 and 2030.
Total employment impacts by channel, gross and net, in 2020, 2025, and 2030
Gains | Losses | Net | |||||||||
---|---|---|---|---|---|---|---|---|---|---|---|
2020 | 2025 | 2030 | 2020 | 2025 | 2030 | 2020 | 2025 | 2030 | |||
Direct | 119,938 | 83,059 | 79,552 | 24,263 | 52,959 | 64,672 | 95,675 | 30,100 | 14,880 | ||
Indirect | 353,210 | 257,778 | 239,342 | 88,656 | 181,526 | 229,880 | 264,554 | 76,252 | 9,462 | ||
Supplier | 136,504 | 99,634 | 92,687 | 29,117 | 62,884 | 80,644 | 107,387 | 36,750 | 12,042 | ||
K-services | 60,433 | 41,848 | 36,268 | 29,713 | 54,273 | 68,722 | 30,720 | -12,424 | -32,453 | ||
Induced, direct | 72,723 | 55,477 | 54,058 | 13,237 | 28,358 | 34,056 | 59,486 | 27,118 | 20,002 | ||
Induced, indirect | 72,664 | 52,718 | 48,639 | 14,511 | 31,528 | 40,849 | 58,153 | 21,190 | 7,789 | ||
Federal | 3,230 | 2,404 | 2,281 | 616 | 1,330 | 1,664 | 2,613 | 1,073 | 617 | ||
State/local | 7,656 | 5,697 | 5,408 | 1,461 | 3,154 | 3,944 | 6,195 | 2,544 | 1,463 | ||
Direct and indirect | 473,147 | 340,837 | 318,894 | 112,918 | 234,486 | 294,552 | 360,229 | 106,352 | 24,342 | ||
Job impact of prices | 0 | 0 | 0 | 75,000 | 0 | 0 | -75,000 | 0 | 0 | ||
Total | 473,147 | 340,837 | 318,894 | 187,918 | 234,486 | 294,552 | 285,229 | 106,352 | 24,342 |
Source: Author's analysis of tables 1 through 3
Sensitivity check on energy efficiency jobs and full-time equivalents
The EPA RIA’s estimates of jobs supported by energy efficiency investments include a caution that these are not expressed as full-time equivalents, while the other direct job flows are. This could potentially skew upward the estimate of jobs supported through these investments. My primary estimate of employment changes has not adjusted the overall numbers for this caution, mostly because the gap between total employment and full-time equivalent employment in sectors heavily represented in energy efficiency investments (mostly manufacturing and construction) is very small. However, I did experiment with adjusting the jobs supported by energy efficiency investments downward by the economy-wide ratio of full-time equivalents to overall employment, with the results shown in Appendix Table A5. This adjustment leads to a roughly 10 percent reduction in jobs supported by energy efficiency investments, which in turn leads to direct and indirect job gains in 2020 falling to approximately 330,000 (down from 360,000 reported in earlier results), and leads to small net job losses in these categories by 2030 (less than 15,000).
It is worth noting that in a well-functioning economy (i.e., one without substantial degrees of economic slack and one no longer stuck in the liquidity trap that has characterized much of the past six years in the American economy), any significant impact on economy-wide employment—either positive or negative—would likely be met by a countervailing response from the Federal Reserve. In a sense, the Fed’s job is precisely to make sure that the economy-wide employment response to any shock like the CPP is zero. However, the Fed’s countervailing response may be imperfect, and it is useful to know the direction in which the Fed will have to push the economy following the implementation of the CPP. And, as I will explain, the geographic distribution of gains and losses means that even if the Fed were to fully neutralize the national employment impacts of the CPP, impacts that differ across regions would remain.
Comparison of job composition of gaining versus losing industries
In addition to changes in employment levels, policymakers may also be interested in changes in the composition of jobs spurred by labor market responses to the CPP. This section combines information from the BLS ERM and demographic and labor market data from the Current Population Survey (CPS) to predict the characteristics of workers that will populate the jobs either displaced or created by the CPP.
I use the CPS to estimate the share of each industry’s workforce by gender, race, educational attainment, union status, and wage level. I then multiply these shares by the total number of jobs displaced or created by the CPP. I present the results separately for gaining and losing industries in Tables 5 and 6.
The broad summary of differences in job composition between gaining and losing industries can be summarized briefly: Losing industries tend to have fewer workers with a four-year college degree or more education (20.8 percent versus 29.8 percent in gaining industries) and yet have fewer low-wage and more middle-wage jobs. This is likely in part because jobs in Iosing industries are significantly more unionized than in gaining industries (19.8 percent versus 9.0 percent). Jobs in both gaining and losing industries have higher shares of male workers and white workers than economy-wide averages.
Demographic, labor force, and job-quality characteristics of jobs in losing industries
Jobs lost | Percentage of jobs lost | Economy-wide average | |||||||||
---|---|---|---|---|---|---|---|---|---|---|---|
Direct | Supplier | Direct +Supplier | K-input | Total | Direct | Supplier | Direct +Supplier | K-input | Total | ||
Totals | 24,263 | 29,117 | 53,379 | 29,115 | 82,494 | 29.4% | 35.3% | 64.7% | 35.3% | 100.0% | 100.0% |
Gender | |||||||||||
Male | 21,034 | 24,665 | 45,699 | 24,986 | 70,685 | 86.7% | 84.7% | 85.6% | 85.8% | 85.7% | 51.5% |
Female | 3,229 | 4,452 | 7,681 | 4,129 | 11,809 | 13.3% | 15.3% | 14.4% | 14.2% | 14.3% | 48.5 |
Race | |||||||||||
Non-Hispanic white | 20,907 | 24,521 | 45,428 | 19,295 | 64,724 | 86.2% | 84.2% | 85.1% | 66.3% | 78.5% | 66.2% |
Non-Hispanic black | 1,210 | 1,748 | 2,958 | 1,608 | 4,566 | 5.0% | 6.0% | 5.5% | 5.5% | 5.5% | 10.9 |
Hispanic | 1,315 | 1,842 | 3,158 | 6,658 | 9,816 | 5.4% | 6.3% | 5.9% | 22.9% | 11.9% | 15.8 |
Asian (including Pacific islander) | 224 | 342 | 566 | 1,131 | 1,697 | 0.9% | 1.2% | 1.1% | 3.9% | 2.1% | 5.3 |
Other | 606 | 664 | 1,270 | 422 | 1,691 | 2.5% | 2.3% | 2.4% | 1.4% | 2.1% | 1.7 |
Age | |||||||||||
Less than 25 years | 1,760 | 1,978 | 3,738 | 2,768 | 6,506 | 7.3% | 6.8% | 7.0% | 9.5% | 7.9% | 14.6% |
25–54 | 17,941 | 21,668 | 39,609 | 22,273 | 61,881 | 73.9% | 74.4% | 74.2% | 76.5% | 75.0% | 70.0 |
55 years and older | 4,562 | 5,471 | 10,032 | 4,074 | 14,107 | 18.8% | 18.8% | 18.8% | 14.0% | 17.1% | 15.4 |
Union status | |||||||||||
Covered | 5,559 | 7,025 | 12,585 | 3,757 | 16,342 | 22.9% | 24.1% | 23.6% | 12.9% | 19.8% | 10.7% |
Not covered | 18,703 | 22,091 | 40,795 | 25,358 | 66,153 | 77.1% | 75.9% | 76.4% | 87.1% | 80.2% | 89.3 |
Education | |||||||||||
Less than high school | 1,140 | 1,185 | 2,325 | 4,818 | 7,143 | 4.7% | 4.1% | 4.4% | 16.5% | 8.7% | 9.7% |
High school only | 10,817 | 11,815 | 22,632 | 11,024 | 33,656 | 44.6% | 40.6% | 42.4% | 37.9% | 40.8% | 28.2% |
Some college | 7,539 | 9,409 | 16,949 | 7,543 | 24,492 | 31.1% | 32.3% | 31.8% | 25.9% | 29.7% | 29.8% |
Bachelor’s only | 3,509 | 4,937 | 8,447 | 4,278 | 12,724 | 14.5% | 17.0% | 15.8% | 14.7% | 15.4% | 21.4% |
Advanced degree | 1,257 | 1,770 | 3,027 | 1,453 | 4,480 | 5.2% | 6.1% | 5.7% | 5.0% | 5.4% | 11.0% |
Wage quintile | |||||||||||
First (lowest) | 714 | 853 | 1,567 | 2,602 | 4,169 | 2.9% | 2.9% | 2.9% | 8.9% | 5.1% | 20.5% |
Second | 1,944 | 2,317 | 4,261 | 5,241 | 9,503 | 8.0% | 8.0% | 8.0% | 18.0% | 11.5% | 19.6% |
Third | 4,032 | 4,804 | 8,836 | 6,873 | 15,709 | 16.6% | 16.5% | 16.6% | 23.6% | 19.0% | 20.0% |
Fourth | 9072 | 10291 | 19363 | 7524 | 26887 | 37.4% | 35.3% | 36.3% | 25.8% | 32.6% | 20.0% |
Fifth (highest) | 8502 | 10851 | 19352 | 6875 | 26227 | 35.0% | 37.3% | 36.3% | 23.6% | 31.8% | 20.0% |
Note: Job estimates do not include spending effects. Employment statistics represent pooled data from 2009–2012. Figure sums relayed in the text may vary slightly from the table due to rounding.
Source: Author’s analysis of EPA Regulatory Impact Analysis of Clean Power Plan (2014), Bureau of Labor Statistics' Employment Requirements Matrices (ERMs), and Current Population Survey (CPS) public data series
Demographic, labor force, and job-quality characteristics of jobs in gaining industries
Jobs gained | Percentage of jobs gained | Economy-wide average | |||||||||
---|---|---|---|---|---|---|---|---|---|---|---|
Direct | Supplier | Direct +Supplier | K-input | Total | Direct | Supplier | Direct +Supplier | K-input | Total | ||
Totals | 119,938 | 136,504 | 256,441 | 59,969 | 316,410 | 37.9% | 43.1% | 81.0% | 19.0% | 100.0% | 100.0% |
Gender | |||||||||||
Male | 87,464 | 99,434 | 186,898 | 51,464 | 238,363 | 72.9% | 72.8% | 72.9% | 85.8% | 75.3% | 51.5% |
Female | 32,474 | 37,069 | 69,543 | 8,504 | 78,047 | 27.1% | 27.2% | 27.1% | 14.2% | 24.7% | 48.5% |
Race | |||||||||||
Non-Hispanic white | 84,543 | 99,750 | 184,293 | 39,743 | 224,036 | 70.5% | 73.1% | 71.9% | 66.3% | 70.8% | 66.2% |
Non-Hispanic black | 9,259 | 11,067 | 20,326 | 3,313 | 23,639 | 7.7% | 8.1% | 7.9% | 5.5% | 7.5% | 10.9% |
Hispanic | 17,808 | 15,792 | 33,600 | 13,714 | 47,314 | 14.8% | 11.6% | 13.1% | 22.9% | 15.0% | 15.8% |
Asian (including Pacific islander) | 6,700 | 8,048 | 14,748 | 2,330 | 17,078 | 5.6% | 5.9% | 5.8% | 3.9% | 5.4% | 5.3% |
Other | 1,627 | 1,848 | 3,475 | 869 | 4,344 | 1.4% | 1.4% | 1.4% | 1.4% | 1.4% | 1.7% |
Age | |||||||||||
Less than 25 years | 9,135 | 9,367 | 18,502 | 5,701 | 24,203 | 7.6% | 6.9% | 7.2% | 9.5% | 7.6% | 14.6% |
25–54 | 89,897 | 101,761 | 191,658 | 45,875 | 237,533 | 75.0% | 74.5% | 74.7% | 76.5% | 75.1% | 70.0% |
55 years and older | 20,905 | 25,376 | 46,281 | 8,392 | 54,673 | 17.4% | 18.6% | 18.0% | 14.0% | 17.3% | 15.4% |
Union status | |||||||||||
Covered | 9,847 | 11,034 | 20,880 | 7,739 | 28,619 | 8.2% | 8.1% | 8.1% | 12.9% | 9.0% | 10.7% |
Not covered | 110,091 | 125,470 | 235,561 | 52,230 | 287,791 | 91.8% | 91.9% | 91.9% | 87.1% | 91.0% | 89.3% |
Education | |||||||||||
Less than high school | 11,201 | 8,911 | 20,112 | 9,923 | 30,035 | 9.3% | 6.5% | 7.8% | 16.5% | 9.5% | 9.7% |
High school only | 38,404 | 43,819 | 82,223 | 22,706 | 104,929 | 32.0% | 32.1% | 32.1% | 37.9% | 33.2% | 28.2% |
Some college | 32,686 | 38,874 | 71,560 | 15,536 | 87,096 | 27.3% | 28.5% | 27.9% | 25.9% | 27.5% | 29.8% |
Bachelor’s only | 25,780 | 30,394 | 56,173 | 8,811 | 64,984 | 21.5% | 22.3% | 21.9% | 14.7% | 20.5% | 21.4% |
Advanced degree | 11,867 | 14,506 | 26,373 | 2,993 | 29,366 | 9.9% | 10.6% | 10.3% | 5.0% | 9.3% | 11.0% |
Wage quintile | |||||||||||
First (lowest) | 10,609 | 9,311 | 19,921 | 5,360 | 25,281 | 8.8% | 6.8% | 7.8% | 8.9% | 8.0% | 20.5% |
Second | 19,151 | 19,837 | 38,989 | 10,796 | 49,785 | 16.0% | 14.5% | 15.2% | 18.0% | 15.7% | 19.6% |
Third | 26,581 | 30,669 | 57,251 | 14,156 | 71,407 | 22.2% | 22.5% | 22.3% | 23.6% | 22.6% | 20.0% |
Fourth | 31,151 | 37,126 | 68,277 | 15,496 | 83,774 | 26.0% | 27.2% | 26.6% | 25.8% | 26.5% | 20.0% |
Fifth (highest) | 32,444 | 39,560 | 72,004 | 14,160 | 86,164 | 27.1% | 29.0% | 28.1% | 23.6% | 27.2% | 20.0% |
Note: Job estimates do not include spending effects. Employment statistics represent pooled data from 2009–2012. Figure sums relayed in the text may vary slightly from the table due to rounding.
Source: Author’s analysis of EPA Regulatory Impact Analysis of Clean Power Plan (2014), Bureau of Labor Statistics' Employment Requirements Matrices (ERMs), and Current Population Survey (CPS) public data series
Specific challenges posed by transition from losing industries
These indicators of job quality highlight some of the key challenges in managing the labor market transitions that are likely to result from the CPP. Specifically, workers displaced by the CPP will tend to have less formal credentials than economy-wide averages and also skew older. Both of these characteristics correlate with lower re-employment probabilities and lower quality jobs when alternative employment is secured (Sum et al. 2011). Further, because jobs in losing industries pay higher than average wages even for a workforce that has fewer formal educational credentials, the expected wage loss from displacement from these industries is expected to be higher.
Another transition issue comes from the disproportionate impact of job losses due to price effects on energy-intensive, trade-exposed industries. As Figure B shows, there are a small number of manufacturing industries that have significantly higher energy cost shares than others, and so these industries may see a significant decline in their international competitive position if domestic policy (i.e., the CPP) were to make electricity significantly more expensive for them relative to the global competition.
Energy costs as a share of gross output
1 | 0.22324% |
2 | 0.22611% |
3 | 0.35292% |
4 | 0.37889% |
5 | 0.39347% |
6 | 0.40630% |
7 | 0.42161% |
8 | 0.43271% |
9 | 0.44781% |
10 | 0.45624% |
11 | 0.49416% |
12 | 0.50240% |
13 | 0.51525% |
14 | 0.52217% |
15 | 0.52571% |
16 | 0.52634% |
17 | 0.53448% |
18 | 0.53555% |
19 | 0.55027% |
20 | 0.55063% |
21 | 0.55291% |
22 | 0.56069% |
23 | 0.56071% |
24 | 0.56497% |
25 | 0.56674% |
26 | 0.57577% |
27 | 0.60209% |
28 | 0.60612% |
29 | 0.61000% |
30 | 0.61064% |
31 | 0.61504% |
32 | 0.61527% |
33 | 0.61989% |
34 | 0.62021% |
35 | 0.62253% |
36 | 0.62582% |
37 | 0.62790% |
38 | 0.63885% |
39 | 0.64145% |
40 | 0.64161% |
41 | 0.64289% |
42 | 0.64490% |
43 | 0.65228% |
44 | 0.66453% |
45 | 0.66700% |
46 | 0.67621% |
47 | 0.68092% |
48 | 0.68128% |
49 | 0.68468% |
50 | 0.68818% |
51 | 0.68845% |
52 | 0.69172% |
53 | 0.69226% |
54 | 0.69276% |
55 | 0.69281% |
56 | 0.69625% |
57 | 0.70396% |
58 | 0.71109% |
59 | 0.71126% |
60 | 0.71268% |
61 | 0.71379% |
62 | 0.71397% |
63 | 0.71487% |
64 | 0.71625% |
65 | 0.71632% |
66 | 0.71966% |
67 | 0.72418% |
68 | 0.72494% |
69 | 0.72730% |
70 | 0.73003% |
71 | 0.73502% |
72 | 0.73506% |
73 | 0.73748% |
74 | 0.74012% |
75 | 0.74122% |
76 | 0.74297% |
77 | 0.74310% |
78 | 0.74529% |
79 | 0.74585% |
80 | 0.75029% |
81 | 0.75927% |
82 | 0.76105% |
83 | 0.76426% |
84 | 0.76854% |
85 | 0.76867% |
86 | 0.77157% |
87 | 0.77829% |
88 | 0.78043% |
89 | 0.78156% |
90 | 0.78496% |
91 | 0.78529% |
92 | 0.78753% |
93 | 0.78792% |
94 | 0.79243% |
95 | 0.79302% |
96 | 0.79318% |
97 | 0.79379% |
98 | 0.79486% |
99 | 0.79796% |
100 | 0.79849% |
101 | 0.80669% |
102 | 0.80862% |
103 | 0.81048% |
104 | 0.81182% |
105 | 0.81307% |
106 | 0.82399% |
107 | 0.82545% |
108 | 0.82809% |
109 | 0.83253% |
110 | 0.83748% |
111 | 0.83763% |
112 | 0.83946% |
113 | 0.84108% |
114 | 0.84279% |
115 | 0.85208% |
116 | 0.85333% |
117 | 0.85948% |
118 | 0.85989% |
119 | 0.86898% |
120 | 0.86948% |
121 | 0.87647% |
122 | 0.87977% |
123 | 0.88181% |
124 | 0.88205% |
125 | 0.88358% |
126 | 0.88370% |
127 | 0.88512% |
128 | 0.88515% |
129 | 0.88574% |
130 | 0.89583% |
131 | 0.89826% |
132 | 0.89834% |
133 | 0.90510% |
134 | 0.90750% |
135 | 0.90769% |
136 | 0.91329% |
137 | 0.91383% |
138 | 0.91449% |
139 | 0.91462% |
140 | 0.91559% |
141 | 0.91631% |
142 | 0.91728% |
143 | 0.91856% |
144 | 0.91899% |
145 | 0.92179% |
146 | 0.92299% |
147 | 0.92539% |
148 | 0.92648% |
149 | 0.92790% |
150 | 0.93005% |
151 | 0.93541% |
152 | 0.93686% |
153 | 0.93722% |
154 | 0.94030% |
155 | 0.94099% |
156 | 0.94120% |
157 | 0.94267% |
158 | 0.94329% |
159 | 0.94459% |
160 | 0.95215% |
161 | 0.95468% |
162 | 0.95774% |
163 | 0.95984% |
164 | 0.97353% |
165 | 0.97540% |
166 | 0.97661% |
167 | 0.97714% |
168 | 0.97865% |
169 | 0.98275% |
170 | 0.98506% |
171 | 0.98776% |
172 | 0.99165% |
173 | 0.99272% |
174 | 0.99313% |
175 | 0.99326% |
176 | 0.99475% |
177 | 0.99592% |
178 | 1.00180% |
179 | 1.00643% |
180 | 1.00861% |
181 | 1.00963% |
182 | 1.00978% |
183 | 1.01450% |
184 | 1.03551% |
185 | 1.03605% |
186 | 1.03642% |
187 | 1.03867% |
188 | 1.03900% |
189 | 1.04108% |
190 | 1.04240% |
191 | 1.04610% |
192 | 1.04973% |
193 | 1.05332% |
194 | 1.06016% |
195 | 1.06212% |
196 | 1.06363% |
197 | 1.06778% |
198 | 1.06948% |
199 | 1.07260% |
200 | 1.07382% |
201 | 1.07659% |
202 | 1.07669% |
203 | 1.07858% |
204 | 1.08265% |
205 | 1.09154% |
206 | 1.09245% |
207 | 1.09663% |
208 | 1.10045% |
209 | 1.10054% |
210 | 1.10595% |
211 | 1.11598% |
212 | 1.12065% |
213 | 1.12267% |
214 | 1.12402% |
215 | 1.12996% |
216 | 1.13132% |
217 | 1.13192% |
218 | 1.13537% |
219 | 1.13588% |
220 | 1.14663% |
221 | 1.17156% |
222 | 1.17506% |
223 | 1.17535% |
224 | 1.18224% |
225 | 1.18249% |
226 | 1.18759% |
227 | 1.19236% |
228 | 1.19670% |
229 | 1.19771% |
230 | 1.19946% |
231 | 1.21657% |
232 | 1.21855% |
233 | 1.22146% |
234 | 1.23628% |
235 | 1.24558% |
236 | 1.24620% |
237 | 1.24734% |
238 | 1.24774% |
239 | 1.25315% |
240 | 1.25479% |
241 | 1.25821% |
242 | 1.26951% |
243 | 1.28280% |
244 | 1.28640% |
245 | 1.28963% |
246 | 1.29731% |
247 | 1.30077% |
248 | 1.30352% |
249 | 1.30735% |
250 | 1.30874% |
251 | 1.30906% |
252 | 1.30973% |
253 | 1.31145% |
254 | 1.31156% |
255 | 1.31195% |
256 | 1.31610% |
257 | 1.33060% |
258 | 1.33422% |
259 | 1.34219% |
260 | 1.34368% |
261 | 1.34810% |
262 | 1.35273% |
263 | 1.35476% |
264 | 1.36137% |
265 | 1.36936% |
266 | 1.37651% |
267 | 1.39308% |
268 | 1.39467% |
269 | 1.39805% |
270 | 1.40017% |
271 | 1.40029% |
272 | 1.40988% |
273 | 1.40991% |
274 | 1.42012% |
275 | 1.42632% |
276 | 1.43301% |
277 | 1.43761% |
278 | 1.44063% |
279 | 1.44931% |
280 | 1.45222% |
281 | 1.46052% |
282 | 1.46269% |
283 | 1.46324% |
284 | 1.46810% |
285 | 1.47328% |
286 | 1.48356% |
287 | 1.48445% |
288 | 1.48765% |
289 | 1.49797% |
290 | 1.50029% |
291 | 1.50563% |
292 | 1.51668% |
293 | 1.52490% |
294 | 1.53302% |
295 | 1.53846% |
296 | 1.54792% |
297 | 1.55145% |
298 | 1.55735% |
299 | 1.56027% |
300 | 1.56134% |
301 | 1.59264% |
302 | 1.59375% |
303 | 1.59509% |
304 | 1.59593% |
305 | 1.61729% |
306 | 1.61742% |
307 | 1.61956% |
308 | 1.65079% |
309 | 1.66238% |
310 | 1.67698% |
311 | 1.67787% |
312 | 1.68092% |
313 | 1.68646% |
314 | 1.69088% |
315 | 1.73818% |
316 | 1.75070% |
317 | 1.76083% |
318 | 1.76596% |
319 | 1.77327% |
320 | 1.78299% |
321 | 1.78956% |
322 | 1.79394% |
323 | 1.79932% |
324 | 1.80073% |
325 | 1.80975% |
326 | 1.82039% |
327 | 1.85128% |
328 | 1.86397% |
329 | 1.87180% |
330 | 1.90339% |
331 | 1.91297% |
332 | 1.91788% |
333 | 1.94243% |
334 | 1.94646% |
335 | 1.96773% |
336 | 1.97934% |
337 | 2.00049% |
338 | 2.00272% |
339 | 2.02367% |
340 | 2.02407% |
341 | 2.05589% |
342 | 2.09201% |
343 | 2.09652% |
344 | 2.11671% |
345 | 2.13663% |
346 | 2.13969% |
347 | 2.16413% |
348 | 2.17914% |
349 | 2.17942% |
350 | 2.18634% |
351 | 2.21503% |
352 | 2.23174% |
353 | 2.25234% |
354 | 2.25474% |
355 | 2.25494% |
356 | 2.27897% |
357 | 2.28444% |
358 | 2.28560% |
359 | 2.28634% |
360 | 2.30268% |
361 | 2.31528% |
362 | 2.32080% |
363 | 2.33085% |
364 | 2.33738% |
365 | 2.33998% |
366 | 2.34653% |
367 | 2.36487% |
368 | 2.42850% |
369 | 2.44608% |
370 | 2.47917% |
371 | 2.48971% |
372 | 2.50601% |
373 | 2.56083% |
374 | 2.57335% |
375 | 2.62798% |
376 | 2.68093% |
377 | 2.68691% |
378 | 2.69049% |
379 | 2.69778% |
380 | 2.75056% |
381 | 2.75235% |
382 | 2.76223% |
383 | 2.77283% |
384 | 2.81731% |
385 | 2.87016% |
386 | 2.87480% |
387 | 2.90541% |
388 | 2.96488% |
389 | 2.98746% |
390 | 2.99783% |
391 | 3.06944% |
392 | 3.07280% |
393 | 3.11001% |
394 | 3.18776% |
395 | 3.27978% |
396 | 3.28111% |
397 | 3.36815% |
398 | 3.38491% |
399 | 3.40537% |
400 | 3.44735% |
401 | 3.65805% |
402 | 3.66780% |
403 | 3.67948% |
404 | 3.68478% |
405 | 3.78927% |
406 | 3.86233% |
407 | 3.88671% |
408 | 3.89086% |
409 | 3.98395% |
410 | 4.05484% |
411 | 4.13173% |
412 | 4.14306% |
413 | 4.15919% |
414 | 4.18434% |
415 | 4.18845% |
416 | 4.19838% |
417 | 4.43143% |
418 | 4.53024% |
419 | 4.67734% |
420 | 4.79830% |
421 | 5.11630% |
422 | 5.11902% |
423 | 5.12252% |
424 | 5.15962% |
425 | 5.26967% |
426 | 5.29506% |
427 | 5.63911% |
428 | 5.70097% |
429 | 5.81583% |
430 | 5.90118% |
431 | 5.92304% |
432 | 5.95768% |
433 | 6.01956% |
434 | 6.07581% |
435 | 6.07775% |
436 | 6.20158% |
437 | 6.28326% |
438 | 6.99019% |
439 | 6.99720% |
440 | 7.01184% |
441 | 7.13364% |
442 | 7.21870% |
443 | 7.37739% |
444 | 7.48520% |
445 | 7.84048% |
446 | 8.47130% |
447 | 8.85738% |
448 | 8.92859% |
449 | 9.73970% |
450 | 9.83184% |
451 | 12.14270% |
452 | 12.37214% |
453 | 13.49690% |
454 | 14.08653% |
455 | 18.28887% |
456 | 18.30725% |
457 | 18.53940% |
458 | 20.64040% |
459 | 23.05939% |
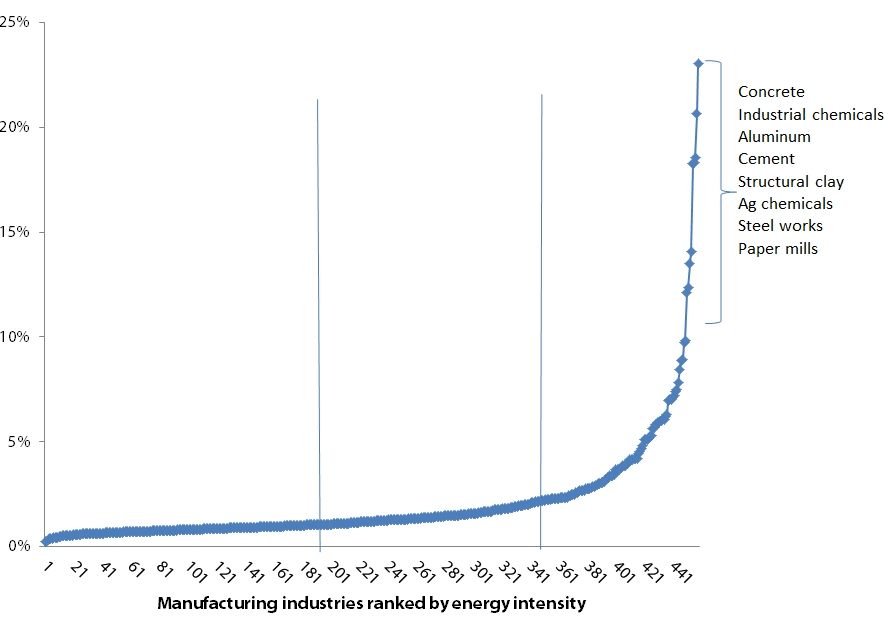
Source: Industry Productivity Database from the National Bureau of Economic Research
I first examine whether manufacturing overall bears a disproportionate share of job losses stemming from price increases. Appendix Table A6 shows the results of the state/panel regressions examined earlier, but now with manufacturing employment as the dependent variable. In the preferred specification (column 2), the coefficient on electricity prices is larger than for overall employment (0.03 versus 0.017) and is statistically significant. Applying this coefficient result to the expected price change resulting from the CPP implies manufacturing job loss of roughly 20,000, or about one-fifth of the entire predicted job losses due to higher prices. Manufacturing employment is far below 10 percent of total employment, so this is clearly a disproportionate effect.
While manufacturing overall bears a disproportionate burden from price increases, this still leaves open the question of how much of this burden stems from eroded international competitiveness. Aldy and Pizer (2014) recently studied how much of output and employment declines stemming from increasing energy prices is the result of declining international competitiveness. Their results for overall manufacturing, as well as for some particularly energy-intensive sectors, are shown in Figure C. For particularly energy-intensive industries, about one-fifth of the entire output and employment decline stemming from higher energy prices is due to an eroded position in international markets.
Share of output declines stemming from higher energy prices attributable to reduced competitiveness
Aluminum | 18% |
---|---|
Cement | 17.1 |
Chemicals | 15.4 |
Paper | 14.2 |
Bulk glass | 13.8 |
Iron and steel | 12.4 |
All industry | 5.1% |
Source: Aldy and Pizer (2014)
Figure D highlights another concern related to transition challenges posed by the CPP. The drivers of job displacements in our analysis are the closure of coal-fired EGUs and the reduction in coal mining. Figure D adds together state employment in mining and a rough estimate of state employment in coal-fired EGUs and divides by total employment in the state, to gain a measure that can be thought of as potential exposure to job losses from the CPP.4 It then plots this measure of potential exposure to job losses against each state’s average per capita personal income. The key finding is that potential exposure to job displacements caused by the CPP seems likely to occur disproportionately in poorer states, which could hence have greater trouble finding resources to deal with the needed transitions. Because of this issue, and because the benefits of mitigating carbon emissions are national (indeed, global), this seems like a strong basis for federal policymakers to act to provide relief for states and communities that will have the largest necessary adjustments stemming from the CPP.
Relationship between potential exposure to job losses from the CPP and state average per capita income
Gap between total employment shares and loss shares | State PIPC | |
---|---|---|
Texas | -8.83% | 98 |
Kentucky | -3.74% | 80.9 |
West Virginia | -3.38% | 79.4 |
Wyoming | -3.25% | 118 |
Oklahoma | -3.09% | 93.5 |
Indiana | -2.61% | 86.3 |
North Dakota | -2.39% | 118.8 |
New Mexico | -1.95% | 80.3 |
Missouri | -1.91% | 90.8 |
Louisiana | -1.76% | 92 |
Alabama | -1.51% | 81.5 |
Colorado | -1.49% | 104.8 |
Utah | -1.18% | 81.8 |
Kansas | -0.93% | 99.2 |
Arizona | -0.84% | 82.6 |
Arkansas | -0.83% | 82 |
Iowa | -0.68% | 100 |
Alaska | -0.61% | 112 |
Nebraska | -0.54% | 105.3 |
Ohio | -0.24% | 91.7 |
Montana | -0.15% | 87.9 |
Michigan | -0.05% | 87.2 |
South Carolina | -0.05% | 80 |
Nevada | -0.04% | 87.6 |
Mississippi | 0.14% | 75.8 |
South Dakota | 0.16% | 102.8 |
Vermont | 0.19% | 101.6 |
North Carolina | 0.22% | 86.4 |
Wisconsin | 0.26% | 96.6 |
Tennessee | 0.27% | 88.4 |
Idaho | 0.27% | 80.7 |
Delaware | 0.32% | 100.1 |
Maine | 0.32% | 91.4 |
Rhode Island | 0.34% | 105 |
New Hampshire | 0.36% | 114 |
Hawaii | 0.45% | 101 |
Georgia | 0.48% | 84.5 |
Minnesota | 0.57% | 106.1 |
Oregon | 0.73% | 89 |
Connecticut | 1.19% | 135.5 |
Virginia | 1.64% | 109.1 |
Washington | 1.90% | 106.6 |
Maryland | 1.91% | 120.2 |
Massachusetts | 2.42% | 127.9 |
Pennsylvania | 2.48% | 103.2 |
New Jersey | 2.83% | 123.7 |
Illinois | 3.24% | 104.9 |
Florida | 3.40% | 92.7 |
New York | 6.32% | 121.7 |
California | 9.67% | 108.2 |
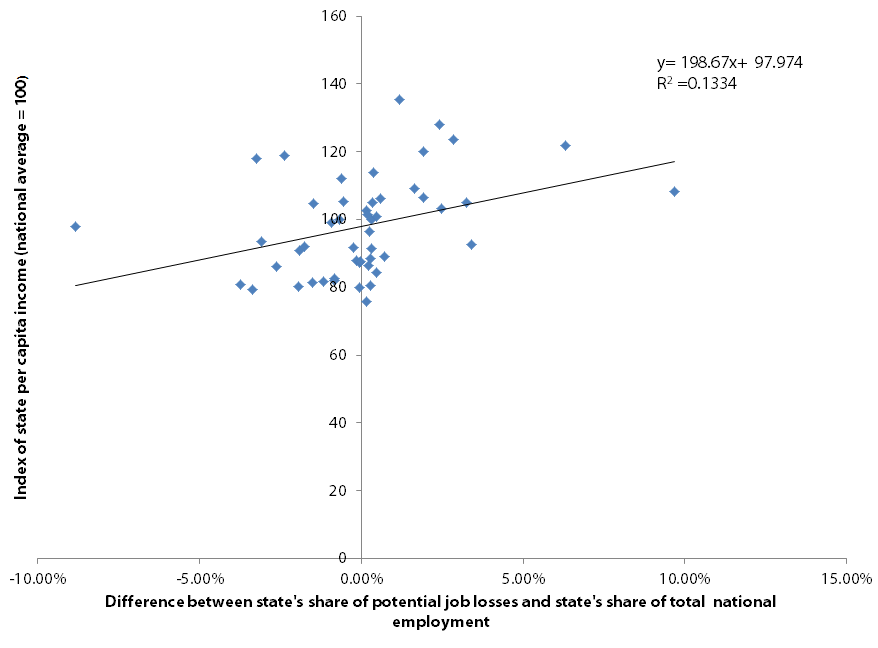
Transition policies
President Obama’s fiscal year 2016 budget proposal includes support for managing the transition to the CPP. This proposal—so far unapproved—included a new $4 billion fund to encourage states to make faster and deeper cuts to emissions from power plants and an additional $2 billion tax credit for power plants that capture their carbon dioxide. These recommended financial supports indicate that the administration acknowledges that a key downside to addressing greenhouse gas mitigation through regulation rather than legislation is that it has the potential to create market distortions that may require additional intervention. A legislative solution would have provided the opportunity to bundle job-creating investments and transition assistance as a combined policy package that would raise the cost of fossil fuel energy production and trigger displacements from “dirty” to “clean” power. The regulatory approach does not offer that same opportunity. The legislative defeat of greenhouse gas mitigation approaches in 2009 made the regulatory track the only available option, and so it is vital that policymakers concerned about jobs and incomes take steps to blunt any economic harm caused by job displacements spurred by the CPP.
There are many such steps that could be taken. Possibly the most important includes ensuring the viability of the health and pensions funds of coal companies. Many retirees rely on this income, and they should not be punished for policy changes that make company pension obligations untenable. Currently the United Mine Workers (UMW) multi-employer pension fund is roughly $1 billion short of being in actuarial balance, driven predominantly by the rapid shrinkage of the current workforce relative to retirees. Another significant blow to the level of the current workforce could be disastrous for the pension fund. The Obama administration’s fiscal year 2016 budget includes transfers to the UMW pension fund through the Pension Benefits Guaranty Corporation to insure the solvency of the plan. The fiscal year 2016 budget also boosts transfers to health plans administered by the UMW to insure their viability.
Another set of tools would aim to ameliorate the decline in industrial competitiveness that could accompany the rule. For example, if other countries undertook measures to raise the price of carbon emissions, this would stem the competitiveness loss. Signing international agreements that raise the cost of greenhouse gas emissions would be an effective policy tool to mitigate the negative effects of labor market transitions stemming from the rule (and would further make the rule more effective in reducing global emissions by stopping carbon-intensive production from simply “leaking” abroad to other countries that do not regulate or price emissions). Until such an international agreement is reached, the United States could unilaterally impose a “border-adjustment” tariff based on the carbon-intensity of the production of imports. Such a tariff would make the global reduction in emissions stemming from the rule larger, would blunt the employment dislocation in the United States caused by the rule, and is in fact necessary for preserving the principle of nondiscrimination in trade relationships.
Conclusion
The CPP is the largest U.S. undertaking to date aimed at mitigating the effects of global climate change. Given the vast importance of global climate change, this means that the impact of the CPP on economic, health, and environmental outcomes is likely to be quite large—and this is indeed what the EPA’s own impact analysis of the CPP shows. Yet much debate about the CPP (and indeed, about nearly all environmental regulations) has focused on the narrower issue of employment changes spurred by the proposed rule. Economic theory suggests that such employment changes are likely to be modest (see Goodstein 1997 and Bivens 2011). This paper offers a comprehensive account of the economic channels through which the rule’s effects could alter U.S. employment. It finds that these effects are relatively modest in the near term, and are more likely to provide a small net boost in employment by 2020. After this date, the net impacts of the rule on employment converge quickly to zero—becoming almost completely insignificant by 2030.
While the proposed rule’s effect on employment levels is small (and positive), the concentration of job dislocations and the composition of jobs in the losing industries suggest that policymakers should consider complementary policies to adjust and to blunt some of the less desirable outcomes of the rule. The clearest virtue to addressing climate change and greenhouse gas emissions through legislation is precisely that such complementary policies can be bundled together with the mechanisms that reduce emissions. This virtue does not accompany the current efforts to limit greenhouse gas emissions through regulation. While a regulatory approach can be effective in achieving the primary target of reducing greenhouse gas emissions, it needs to be supported by policies that will ensure that groups of workers and communities bearing a disproportionate burden of adjustment are fairly compensated.
About the author
Josh Bivens joined the Economic Policy Institute in 2002 and is currently the director of research and policy. His primary areas of research include macroeconomics, social insurance, and globalization. He has authored or co-authored three books (including The State of Working America, 12th Edition) while working at EPI, edited another, and has written numerous research papers, including for academic journals. He appears often in media outlets to offer economic commentary and has testified several times before the U.S. Congress. He earned his Ph.D. from The New School for Social Research.
Endnotes
1. The employment multipliers for all 195 industries are available upon request.
2. Electricity’s share in the total economy is based on data from 2013 using data collected by the Bureau of Economic Analysis (BEA).
3. For translating changes in spending flows and gross domestic product (GDP) into jobs, see Bivens (2011).
4. The labor supply effects of such an electricity price increase are likely to be considerably smaller. The 5 percent increase in electricity prices represents roughly a 0.12 percent reduction in real wages. Typical labor supply elasticities range from 0.1 to 0.3, so this implies a 0.0036 percent reduction in labor supply at most, or roughly 5,400 fewer jobs stemming from workers’ voluntary labor supply decisions.
5. For the estimate of employment in EGUs by state, we allocate nationwide employment in coal-fired plants by each state’s share of national coal-fired electrical generation, using data from the U.S. Energy Information Association (EIA).
References
Aldy, Joseph, and William Pizer. 2014. The Competitiveness Impacts of Climate Change Mitigation Policies. Working paper, March 2014 draft.
Bivens, Josh. 2003. Updated Employment Multipliers for the US Economy. EPI Working Paper No. 268.
Bivens, Josh. 2011. A Life-Saver, Not a Job-Killer: EPA’s Proposed ‘Air Toxics Rule’ Is No Threat to Job Growth. EPI Briefing Paper No. 312.
Bureau of Labor Statistics (U.S. Department of Labor) Employment Requirements Matrix. 2012. “Historical Employment Requirements Tables, 1993–2012.”
Bureau of Labor Statistics (U.S. Department of Labor) Current Employment Statistics program. Various years. Employment, Hours and Earnings—National [database].
Bureau of Labor Statistics (U.S. Department of Labor) Multifactor Productivity program. Various years. Multifactor Industry Productivity [database].
Bureau of Labor Statistics (U.S. Department of Labor) Consumer Price Indexes program. Various years. All Urban Consumers: Chained Consumer Price Index (CPI) [database].
Current Population Survey public data series. Various years. Aggregate data from basic monthly CPS microdata are available from the Bureau of Labor Statistics through three primary channels: as Historical ‘A’ Tables released with the BLS Employment Situation Summary, through the Labor Force Statistics Including the National Unemployment Rate database, and through series reports.
Deschenes, Olivier. 2012. “Climate Policy and Labor Markets” in The Design and Implementation of US Climate Policy, Don Fullerton and Catherine Wolfram, eds. Chicago: University of Chicago Press.
Electric Power Research Institute. 2014. US Energy Efficiency Potential Through 2035.
Environmental Protection Agency (EPA). 2014. Regulatory Impact Analysis for the Proposed Carbon Pollution Guidelines for Existing Power Plants and Emission Standards for Modified and Reconstructed Power Plants.
Goodstein, Eban. 1997. Jobs and the Environment: The Myth of a National Trade-Off. Economic Policy Institute.
Killian, Lutz. 2008. “The Economic Effects of Energy Price Shocks.” Journal of Economic Literature, volume 46(4), 871-909.
Maddala, G. S., R.P. Trost, H. Li, and F. Joutz. 1997. “Estimation of Short-run and Long-run Elasticities of Energy Demand from Panel Data Using Shrinkage Estimators.” Journal of Business & Economic Statistics, 15(1), 90-100.
National Bureau of Economic Research (NBER). 2013. NBER-CES Manufacturing Industry Database.
Pollin, Robert, James Heintz and Heidi Garrett-Peltier. 2009. The Economic Benefits of Investing in Clean Energy: How the economic stimulus program and new legislation can boost US economic growth and employment. Report jointly released by the Political Economic Research Institute and the Center for American Progress.
Sum, Andrew, and Myrkhaylo Trubskyy, Ishwar Khatiwada, Joseph McLaughlin, and Sheila Palma. 2011. The Job Dislocation and Re-employment Experiences of America’s Older Workers During the Great Recessionary Period of 2007-2009: The Economic Consequences for the Dislocated and the Rest of Society. Center for Labor Market Studies report prepared for Senior Service America
U.S Energy Information Administration (EIA) – Independent Statistics and Analysis. Various Years. State Energy Data System (SEDS) [databases].
Wei, Max, Shana Patadia, and Daniel M. Kammen. 2010. “Putting Renewables and Energy Efficiency to Work: How Many Jobs Can the Clean Energy Industry Generate in the US?” Energy Policy 38, no. 2: 919-931.
Appendix A: EPA estimates of job changes by activity
Table A1 reports directly the EPA estimates of employment change by activity spurred by the CPP. It breaks out these employment changes by three different employment flows: (1) construction of new EGUs and heat rate improvements to existing EGUs and energy efficiency investments; (2) operation and maintenance (O&M) employment in the electrical power generating sector; and (3) extraction of fossil fuels. As with Table 1 in the main text, the employment flows shown in Table A1 average the four different estimates provided in the RIA (state versus regional and option 1 versus option 2).
Table A1 provides the averaged estimates for each employment flow (O&M, construction, and extraction) in each year examined by the RIA (2020, 2025, and 2030). In 2020, construction of natural gas generating capacity increases, as does renewable generation, heat-rate improvement investments, and energy efficiency investments. The sum of this short-run construction activity is 123,000 additional jobs relative to the baseline. In later years, however, this pulling forward of natural gas and renewable construction actually depresses construction employment (relative to the baseline) in 2025 and 2030. Energy efficiency investments, conversely, continue to grow through 2030, though at a slower pace.
The large negative impact of the CPP on coal-sector employment is obvious in O&M employment. O&M employment in coal-fired EGUs falls by nearly 20,000 by 2020, and stays about that depressed relative to the baseline all the way through 2030. This is obviously consistent with the significant decline in coal-fired generation identified in Table 1. In the near term, natural gas O&M employment rises, while O&M employment in oil and gas plants falls. Over longer horizons, O&M employment in all fossil fuel generation (including natural gas) falls relative to the baseline. In 2020, the sum total of O&M employment losses is just under 20,000, rising to roughly 24,000 by 2030.
Losses in coal extraction are large and significant in all three years—12,600 in 2020 and rising to 17,300 by 2030. Natural gas extraction employment actually rises slightly in the near term—increasing by 5,050 in 2020 but then falling. By 2030, it is 2,000 jobs lower than the baseline. Again, the CPP pulls forward natural gas-related jobs and leaves them lower in later years.
EPA estimate of direct employment changes under the Clean Power Plan, by generating source and job category, in 2020, 2025, and 2030
2020 | ||||
---|---|---|---|---|
Construction | O&M | Extraction | Total | |
Coal | 0 | -19,400 | -12,600 | -32,000 |
Natural gas | 6,775 | 1,825 | 5,050 | 13,650 |
Oil and gas | 0 | -2,200 | 0 | -2,200 |
Nuclear | 0 | 0 | 0 | 0 |
Hydro | 0 | 0 | 0 | 0 |
Non-hydro renewable | 15,875 | 0 | 0 | 15,875 |
Energy efficiency | 67,900 | 0 | 0 | 67,900 |
Heat-rate improvements | 32,450 | 0 | 0 | 32,450 |
Total | 123,000 | -19,775 | -7,550 | 95,675 |
2025 | ||||
Coal | 0 | -17,800 | -15,300 | -33,100 |
Natural gas | -25,225 | -725 | 2,700 | -23,250 |
Oil and gas | 0 | -1,900 | 0 | -1,900 |
Nuclear | 0 | 0 | 0 | 0 |
Hydro | 0 | 0 | 0 | 0 |
Non-hydro renewable | -5,750 | 0 | 0 | -5,750 |
Energy efficiency | 94,100 | 0 | 0 | 94,100 |
Heat-rate improvements | 0 | 0 | 0 | 0 |
Total | 63,125 | -20,425 | -12,600 | 30,100 |
2030 | ||||
Coal | 0 | -18,950 | -17,300 | -36,250 |
Natural gas | -34,400 | -3,300 | -2,000 | -39,700 |
Oil and gas | 0 | -2,050 | 0 | -2,050 |
Nuclear | 0 | 0 | 0 | 0 |
Hydro | 0 | 0 | 0 | 0 |
Non-hydro renewable | -2,150 | 0 | 0 | -2,150 |
Energy efficiency | 95,030 | 0 | 0 | 95,030 |
Heat-rate improvements | 0 | 0 | 0 | 0 |
Total | 58,480 | -24,300 | -19,300 | 14,880 |
Note: The abbreviation O&M stands for operation and maintenance. The table shows job changes relative to the non-CPP baseline.
Source: EPA Regulatory Impact Analysis of Clean Power Plan (2014)
Appendix B: Consistency check on EPA employment estimates
The information provided in the RIA and summarized in Tables 1 and 2 allows us to undertake a quick consistency check to see if the numbers seem to be in concordance with what employment and generation estimates from other sources indicate. Specifically, from Table 1, we see that coal-fired generation falls by nearly 20 percent by 2020. Coal EGU O&M employment falls by 19,400, according to Table A1.
Table A2 combines data from the Bureau of Labor Statistics (BLS) Current Employment Statistics (CES) and the Energy Information Agency (EIA) to provide a consistency check on these estimates. The BLS CES data indicate that all fossil fuel–generated electrical utility activity in 2013 generated 100,000 jobs. The EIA data indicate that coal-fired EGUS generated a bit under two-thirds of all fossil fuel-generated electricity in 2013. So, if employment fell in strict proportion to generation, this would imply that a 20 percent reduction in coal-fired generation should only see employment losses of roughly 12,000–14,000 jobs. The fact that the CPP RIA instead forecasts losses of nearly 20,000 jobs due to the 20 percent reduction in coal-fired generation implies that coal-fired EGUs—or at least those coal-fired EGUs that are likely to close in response to the CPP—are more labor-intensive than other fossil fuel-generated EGUs.
This same logic holds in reverse for the short-term changes in natural gas generation. The RIA indicates that natural gas-fired EGU generation increases by 15 percent by 2020. EIA estimates indicate that natural gas is roughly one-third of all fossil fuel-generated electricity; so, if employment were to rise in proportion to generation, this would imply an increase in natural gas O&M employment of roughly 5,000 in 2020. The fact that the CPP RIA only forecasts an increase of 2,000 jobs in natural gas O&M indicates that natural gas—or at least the natural gas generation that increases due to the CPP—is less labor intensive than overall fossil fuel generation.
This implicit finding that coal-fired EGU generation is more labor intensive is largely in line with other data. The EIA data show that levelized costs for fixed O&M (which is largely dominated by labor costs) is higher for coal-fired EGUs than for (most) natural gas EGUs. And Wei, Patadia, and Kammen (2010) show that while fixed O&M employment in both coal and natural gas-fired plants is low compared with other forms of generation, coal O&M employment (per unit of generation) is higher than for natural gas.
In short, the data on generation and direct employment impacts from the CPP RIA seem to be roughly plausible (the employment losses/gains are clearly of the same order of magnitude and quite close to overall generation losses/gains), and the implicit relative rankings of labor intensity match other data.
Implied labor intensity of fossil fuel generation
2013 Generation, EIA | Actual 2013 employment (BLS) | Actual 2013 employment (BLS), share of total | |
---|---|---|---|
Total fossil fuels | 67.7% | 100,200 | 60.8% |
Coal | 43.4% | – | |
Natural gas | 23.6% | – | |
Other fossil fuels | 0.7% | – | |
All non-fossil fuels | 32.3% | 64,700 | 39.2% |
Nuclear | 20.0% | – | |
Hydro | 8.0% | – | |
Non-hydro Renewable | 4.2% | – |
Source: Energy Information Agency (EIA) and Bureau of Labor Statistics (BLS)
Appendix C: Mapping EPA employment estimates into specific industries
Table A3 maps the employment changes by economic activity identified in Table A1 for specific industries that we can use to identify indirect impacts. Many of the employment changes identified in Table A1 are quite straightforward to slot into ERM industries. Coal mining job losses enter into ERM industry code 8—Coal Mining. Natural gas extraction gains (in 2020) and subsequent losses (in 2025 and 2030) enter into ERM code 7—Oil and Gas mining. O&M employment changes (both positive and negative) unfortunately (for the sake of precision) all have to be placed in the same ERM industry classification, 12—electric power utilities.
Slightly more complex decisions must be made to determine which industries are the direct recipients of employment flows due to other effects. Energy efficiency, for example, is not the name of a single industry sector in the ERM. To apportion changes due to energy efficiency investments, we used the data provided by the Electric Power Research Institute (EPRI 2014). EPRI estimates the areas with the highest potential for achieving energy efficiency savings in the residential, commercial, and industry sectors. We used the EPRI estimates of potential savings as weights to apportion the spending flow of investments in energy efficiency. For example, in its estimates for the residential sector, EPRI highlights the highest potential savings from the following categories: space cooling, electronics, water heating, lighting, and household appliances. EPRI provides similar estimates for the commercial and industrial sectors. These categories match tightly to existing ERM categories, and I assume that these flows will be proportional to the amount of energy savings achieved through these investments estimated in the EPRI report. If, for example, lighting accounts for 15 percent of energy savings in the residential sector, I apportion 15 percent of employment gains spurred by energy efficiency investments to the sector in the ERM that best approximates this (electrical lighting equipment).
For apportioning employment flows stemming from investments in electricity generation from renewable sources, we drew on estimates from Pollin, Heintz, and Garrett-Peltier (2009), who undertake a detailed analysis of job creation stemming from clean energy production and provide a mapping of industrial spending associated with investment in renewable energy, based on surveys with industry professionals. We used these mappings to assign direct employment flows stemming from renewable generation construction. Both solar and wind generation require construction employment as the single largest input. The remaining inputs constitute a mix of manufactured goods and technical services, as shown in Table A3.
For apportioning employment flows to ERM industry that occur due to construction of natural gas capacity, we assume that one-third of such flows go to construction jobs, one-third to manufacturing of transmission equipment, and one-sixth each to design services and fabricated metals.
Finally, for heat-rate improvements at existing EGUs, we assign the employment flows equally between EGU O&M jobs, ventilation and cooling equipment, power transmission equipment, and scientific and technical services.
Direct employment changes by ERM sector
ERM industry code | ERM industry label | Job change | ||
---|---|---|---|---|
2020 | 2025 | 2030 | ||
EGU O&M plus fuel extraction | ||||
7 | Oil and gas extraction | 5,050 | 2,700 | -2,000 |
8 | Coal mining | -12,600 | -15,300 | -17,300 |
12 | Electric power generation, transmission, and distribution | -19,775 | -20,425 | -24,300 |
Total | -27,325 | -33,025 | -43,600 | |
Energy efficiency investments | ||||
15 | Construction | 9,480 | 13,138 | 13,267 |
67 | HVAC equipment manufacturing | 12,461 | 17,269 | 17,440 |
69 | Engine, turbine, and power transmission equipment manufacturing | 2,384 | 3,303 | 3,336 |
72 | Communications equipment | 551 | 763 | 771 |
77 | Electric lighting manufacturing | 30,388 | 42,114 | 42,530 |
78 | Household appliance manufacturing | 2,624 | 3,637 | 3,673 |
79 | Electrical equipment manufacturing | 2,775 | 3,845 | 3,883 |
136 | Services to buildings and dwellings | 7,238 | 10,031 | 10,130 |
Total | 67,900 | 94,100 | 95,030 | |
Renewable generation investments | ||||
15 | Construction | 4,445 | -1,610 | -602 |
44 | Plastics product manufacturing | 953 | -345 | -129 |
61 | Machine shops: hardware | 1,389 | -503 | -188 |
63 | Fabricated metal | 953 | -345 | -129 |
69 | Engine, turbine, and power transmission equipment manufacturing | 238 | -86 | -32 |
70 | Machinery manufacturing | 2,937 | -1,064 | -398 |
79 | Electrical equipment manufacturing | 1,389 | -503 | -188 |
80 | Other electrical equipment and component manufacturing | 1,627 | -589 | -220 |
126 | Scientific research and development services | 1,945 | -704 | -263 |
Total | 15,875 | -5,750 | -2,150 | |
Heat-rate improvement investments | ||||
12 | Electric power generation, transmission, and distribution | 8,113 | 0 | 0 |
67 | HVAC equipment manufacturing | 8,113 | 0 | 0 |
69 | Engine, turbine, and power transmission equipment manufacturing | 8,113 | 0 | 0 |
125 | Management, scientific, and technical consulting services | 8,113 | 0 | 0 |
Total | 32,450 | 0 | 0 | |
Natural gas generation construction | ||||
15 | Construction | 2,236 | -8,324 | -11,352 |
63 | Fabricated metal | 1,152 | -4,288 | -5,848 |
69 | Engine, turbine, and power transmission equipment manufacturing | 2,236 | -8,324 | -11,352 |
123 | Design services | 1,152 | -4,288 | -5,848 |
Total | 6,775 | -25,225 | -34,400 |
Source: EPA Regulatory Impact Analysis of Clean Power Plan (2014) and the author's analysis described in the text
Appendix D: State by year panel regressions of electricity prices and employment
Table A4 shows the results of a panel regression with the log of state employment as the dependent variable and the log of end-user electricity prices (and other relevant controls) as the independent variables.
Column 1 shows the results from this regression with year and state fixed effects included. Column 2 includes a state-specific time trend. Column 3 includes a measure of the unemployment gap—the difference between a state’s unemployment rate in a given year and the average unemployment rate for that state over the entire sample period.
We note that higher results are gained if one cuts off the sample in 2008 (as is done in columns 4-6), with the coefficient estimates suggesting job losses of over 150,000 in the specification (column 6) that includes all other controls. It is unclear why including the most recent five years of data changes the results so much, but we prefer to include more data rather than less.
Finally, we also ran regressions that used state/industry cells as the unit of analysis. The employment data let us examine 13 separate industrial sectors within states. Despite the larger sample size, the overall coefficient on state/industry employment in this larger panel never achieved statistical significance. Later sections look just at manufacturing employment across states and do find significant and disproportionate job losses in this sector that are correlated with electricity price differences.
Tables A5 and A6 show the employment impacts of higher energy prices and of energy efficient investments adjusted on an economy-wide full-time equivalent basis.
Employment impacts of higher energy prices, state/year panel results
(1) | (2) | (3) | (4) | (5) | (6) | (7) | (8) | (9) | |
State/year panel | -0.014** | -0.038*** | -0.017*** | -0.017* | -0.049*** | -0.026*** | |||
(.008) | (.0054) | (.0044) | (.009) | (.0055) | (.0047) | ||||
State/industry/year panel | -0.049 | -0.044 | -0.024 | ||||||
(.042) | (.058) | (.058) | |||||||
Predicted employment effect, short-run | 81,453 | 221,088 | 98,908 | 98,908 | 285,087 | 151,270 | – | – | – |
Quadratic in year | yes | yes | yes | yes | yes | yes | yes | yes | yes |
Year fixed effecs | yes | yes | yes | yes | yes | yes | yes | yes | yes |
State fixed effects | yes | yes | yes | yes | yes | yes | yes | yes | yes |
State-specific time trend | no | yes | yes | no | yes | yes | no | yes | yes |
Industry fixed effect | – | – | – | – | – | – | yes | yes | yes |
Industry-specific time trend | – | – | – | – | – | – | no | yes | yes |
Unemployment gap | no | no | yes | no | no | yes | no | no | yes |
Years | 1979–2012 | 1979–2012 | 1979–2012 | 1979–2008 | 1979–2008 | 1979–2008 | 1990–2012 | 1990–2012 | 1990–2012 |
Observations | 1,734 | 1,734 | 1,734 | 1,474 | 1,474 | 1,474 | 14,292 | 14,292 | 14,292 |
Note: ***,**,* denote statistical significance at the 1, 5, and 10 percent levels respectively. Standard errors are in parentheses. Dependent variable is the log of state employment, as described in the text.
Source: BLS Current Employment Statistics (CES) and the State Energy Data System (U.S. EIA)
Jobs supported by energy efficiency investments, adjusted on an economy-wide FTE basis
Direct jobs | Total jobs | ||||||||||||
---|---|---|---|---|---|---|---|---|---|---|---|---|---|
Gains, EE Adj for FTE | Losses | Net | Multiplier | ||||||||||
2020 | 2025 | 2030 | 2020 | 2025 | 2030 | 2020 | 2025 | 2030 | 2020 | 2025 | 2030 | ||
Oil and gas extraction | 5,050 | 2,700 | 0 | 0 | 0 | 2,000 | 5,050 | 2,700 | -2,000 | 6.7 | 39,063 | 20,885 | -15,470 |
Coal mining | 0 | 0 | 0 | 12,600 | 15,300 | 17,300 | -12,600 | -15,300 | -17,300 | 2.7 | -46,495 | -56,458 | -63,838 |
Electric power generation, transmission, and distribution | 0 | 0 | 0 | 11,663 | 20,425 | 24,300 | -11,663 | -20,425 | -24,300 | 4.7 | -66,423 | -116,330 | -138,400 |
Construction | 15,213 | 1,890 | -13 | 0 | 0 | 0 | 15,213 | 1,890 | -13 | 1.7 | 41,668 | 5,176 | -36 |
Plastics product manufacturing | 953 | 0 | 0 | 0 | 345 | 129 | 953 | -345 | -129 | 2.4 | 3,227 | -1,169 | -437 |
Machine shops: hardware | 1,389 | 0 | 0 | 0 | 503 | 188 | 1,389 | -503 | -188 | 1.5 | 3,492 | -1,265 | -473 |
Fabricated metal | 2,104 | 0 | 0 | 0 | 4,633 | 5,977 | 2,104 | -4,633 | -5,977 | 2.3 | 6,891 | -15,174 | -19,575 |
HVAC equipment manufacturing | 19,327 | 15,542 | 15,696 | 0 | 0 | 0 | 19,327 | 15,542 | 15,696 | 3.2 | 82,079 | 66,004 | 66,657 |
Engine, turbine, and power transmission equipment manufacturing | 12,732 | -330 | -334 | 0 | 5,107 | 8,048 | 12,732 | -5,438 | -8,382 | 3.8 | 61,158 | -26,120 | -40,264 |
Machinery manufacturing | 2,937 | 0 | 0 | 0 | 1,064 | 398 | 2,937 | -1,064 | -398 | 3.3 | 12,582 | -4,557 | -1,704 |
Communications equipment | 496 | 687 | 694 | 0 | 0 | 0 | 496 | 687 | 694 | 3.4 | 2,189 | 3,034 | 3,064 |
Electric lighting manufacturing | 27,349 | 37,902 | 38,277 | 0 | 0 | 0 | 27,349 | 37,902 | 38,277 | 3.3 | 117,098 | 162,281 | 163,885 |
Household appliance manufacturing | 2,362 | 3,273 | 3,306 | 0 | 0 | 0 | 2,362 | 3,273 | 3,306 | 4.5 | 13,074 | 18,119 | 18,298 |
Electrical equipment manufacturing | 3,886 | 2,958 | 3,307 | 0 | 0 | 0 | 3,886 | 2,958 | 3,307 | 2.3 | 12,662 | 9,636 | 10,774 |
Other electrical equipment and component manufacturing | 1,627 | 0 | 0 | 0 | 589 | 220 | 1,627 | -589 | -220 | 2.7 | 6,001 | -2,174 | -813 |
Design services | 1,152 | 0 | 0 | 0 | 4,288 | 5,848 | 1,152 | -4,288 | -5,848 | 1.4 | 2,818 | -10,492 | -14,308 |
Management, scientific, and technical consulting services | 8,113 | 0 | 0 | 0 | 0 | 0 | 8,113 | 0 | 0 | 1.6 | 21,364 | 0 | 0 |
Scientific research and development services | 1,945 | 0 | 0 | 0 | 704 | 263 | 1,945 | -704 | -263 | 2.3 | 6,444 | -2,334 | -873 |
Services to buildings and dwellings | 6,514 | 9,028 | 9,117 | 0 | 0 | 0 | 6,514 | 9,028 | 9,117 | 1.3 | 15,024 | 20,821 | 21,027 |
Total | 113,148 | 73,649 | 70,049 | 24,263 | 52,959 | 64,672 | 88,885 | 20,690 | 5,377 | 333,915 | 69,885 | -12,486 |
Note: Because the jobs associated with Energy Efficient (EE) investments are not expressed on a full-time equivalent (FTE) basis as are other job numbers in the RIA, they could be skewed upward. If the ratio of FTEs to total employment that holds economy-wide is applied to these jobs as well, this results in a 10 percent reduction in direct employment associated with EE investments. Note that this is likely a high estimated loss, as the FTE/total employment ratio is likely much higher in manufacturing and construction than in economy-wide numbers.
Source: Author’s analysis using data from the EPA RIA of the Clean Power Plan, BLS Employment Requirements Matrix (ERM), BLS Multi-factor Productivity Data Series, and Census Data on government spending and employment.
Employment impacts of higher energy prices – manufacturing industries only (results of state/panel regressions)
Manufacturing | ||
---|---|---|
(1) | (2) | |
State/industry/year panel | -0.15*** | -.03* |
(.04) | (.02) | |
Predicted employment effect, short-run | 97,500 | 19,500 |
Quadratic in year | yes | yes |
Year fixed effects | yes | yes |
State fixed effects | yes | yes |
State-specific time trend | no | yes |
Industry fixed effect | yes | yes |
Industry-specific time trend | no | yes |
Unemployment gap | no | yes |
Years | 1990–2012 | 1990–2012 |
Observations | 1,184 | 1,184 |
Note: ***,**,* denote statistical significance at the 1, 5, and 10 percent levels respectively. Standard errors are in parentheses.
Source: BLS Current Employment Statistics (CES) and the State Energy Data System (SEDS)